Registry Hub#
The Registry Hub centralizes dataset management, experiment tracking, model registry, and test suite monitoring. It is structured into two main workflows:
Dataset Workflow: Upload, preview, and manage datasets used in experiments.
Experiment Workflow: Track, process, and manage experiments, models, and test suites.
Initialize the Panel#
To create and initialize the Registry Hub panel, use:
# Manage the Registry Hub
from modeva.dashboard.api import registry_hub
registry_hub()
Dataset Workflow#
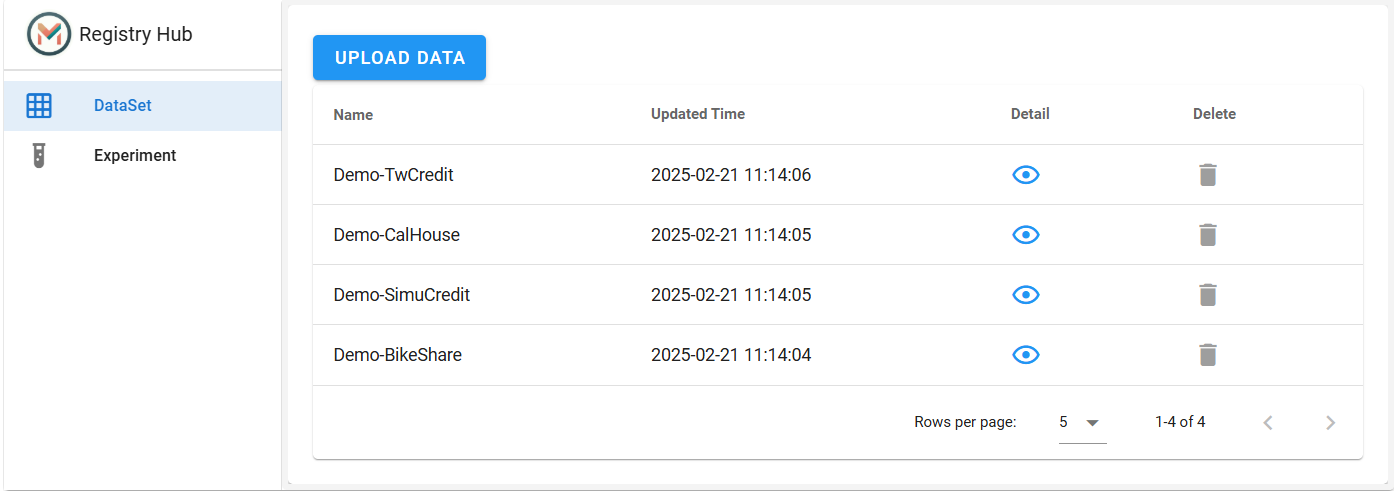
Uploading Data#
Click “Upload Data”.
Select a CSV File: Choose a dataset file (e.g., SimuCredit.csv).
Choose Dataset Type:
Main Dataset: Standalone dataset (e.g., “SimuCredit”).
Extra Dataset: Supplemental data linked to an existing main dataset.
Configure Upload Options:
Dataset Name: Auto-filled from the filename but editable (e.g., “SimuCredit”).
Override: Check to replace an existing dataset with the same name.
Parent Dataset: Required for “Extra” datasets to link to a main dataset.
Click “Register” to finalize.
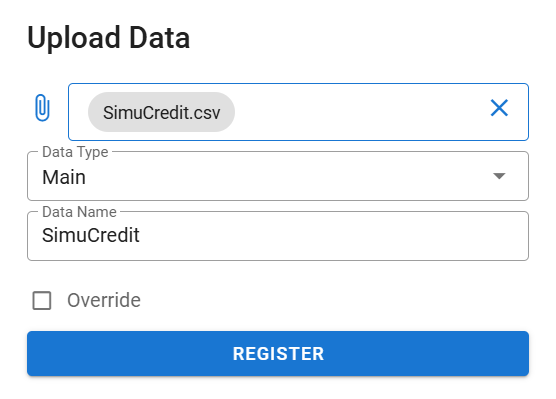
After registration, the dataset appears in the Dataset Registry with columns: Name, Updated Time, Detail, and Delete.
Warning
Uploading a new Main Dataset with Override resets all linked experiments.
Managing Datasets#
All uploaded datasets are listed in the Dataset Registry. There are four datasets stored automatically for demo purposes: Demo-SimuCredit, Demo-BikeShare, Demo-CalHouse, and Demo-TwCredit.
View Details: Click in the Detail column to see metadata like Sample Size (e.g., 20,000 rows for “Demo-SimuCredit”) and splits (main, train, test).
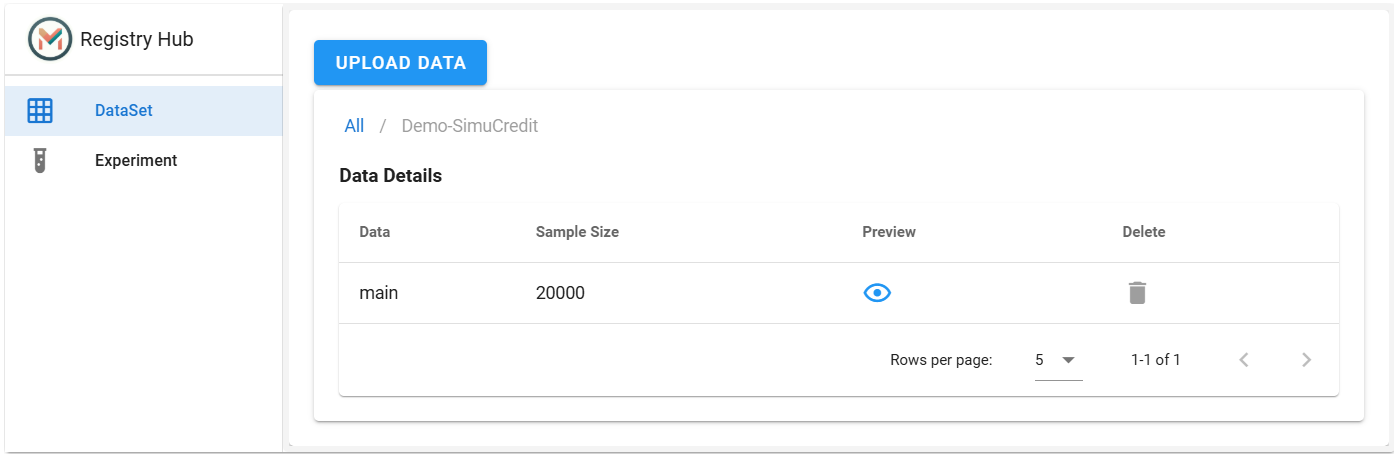
Preview Data: Click in the Preview column to inspect the first 10 rows of the dataset in the View Details tab.
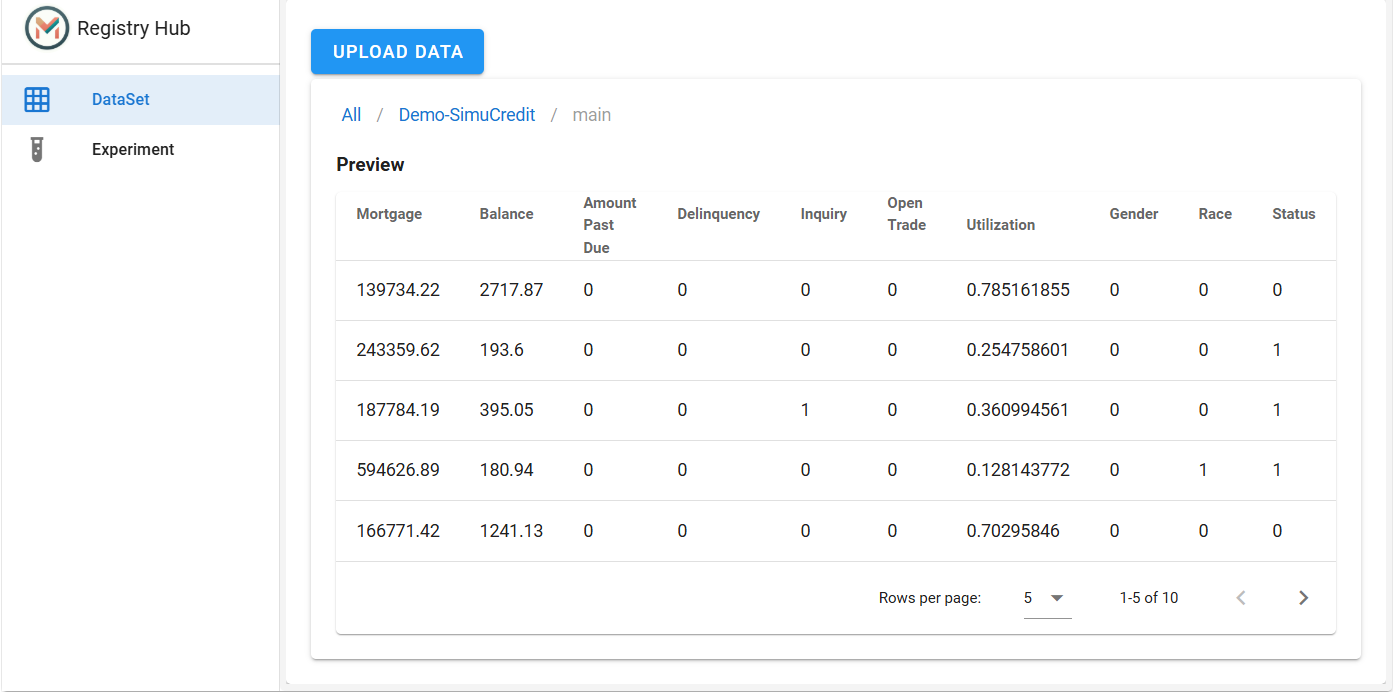
Delete Dataset: Click to remove it. Demo datasets are protected.
Warning
Deleting a dataset, all linked experiments are reset.
Experiment Workflow#
Creating & Managing Experiments#
Create New Experiment: New experiments are created with high code only.
If the experiment name exists, it will load the existing experiment; otherwise, it will create a new experiment.
# Create a new experiment
from modeva import Experiment
exp = Experiment(name='Demo-SimuCredit')
Bind Dataset: Select a dataset (e.g., “Demo-SimuCredit”) to link to the experiment.
# Link the dataset to the experiment
exp.data_load('Demo-SimuCredit')
Clear Experiment: Remove all data and models from the experiment.
# Clear the experiment
exp.clear()
Track Experiments#
Experiments track ML processes and are linked to datasets.
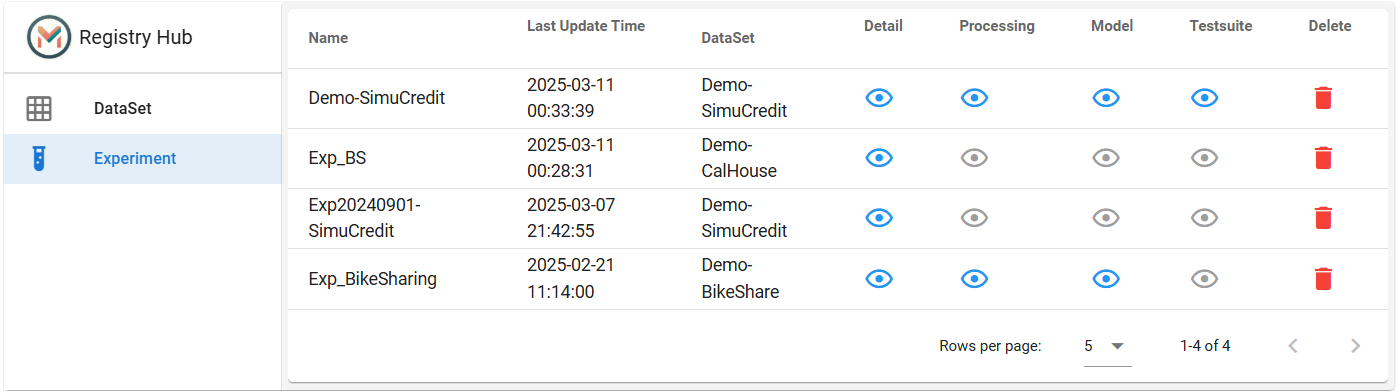
View Experiment Details: Click in the Detail column to see the sample size of each data split, main (20,000), train (16,000), test (4,000).
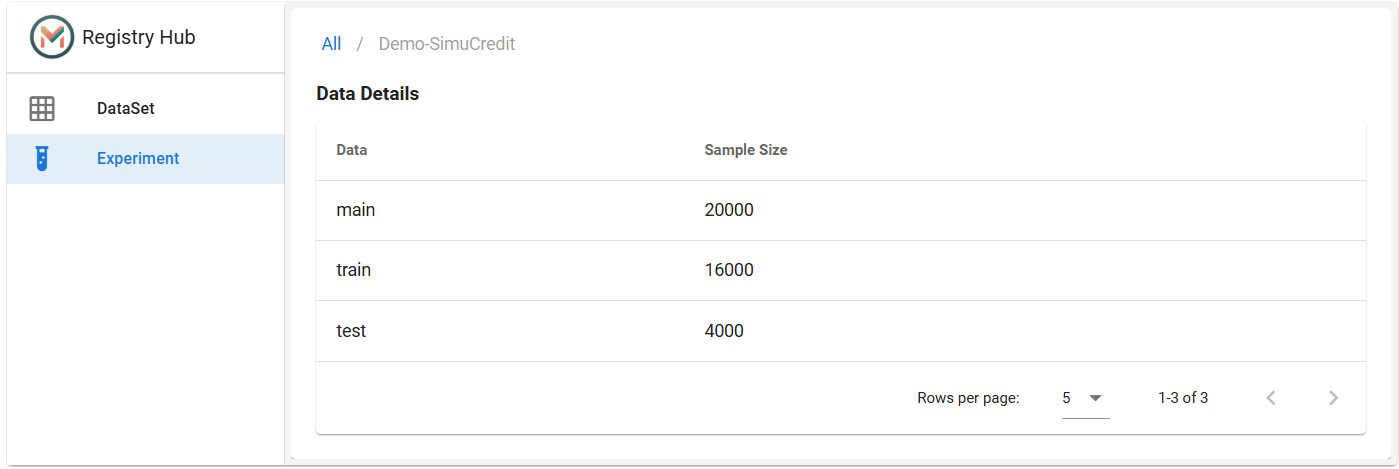
View Processing Steps: Click in the Processing column to see feature-specific transformation records (e.g., scaling, encoding).
Each row represents a feature (e.g., “Utilization”, “Gender”).
Each column represents a feature status (e.g., “Imputation”, “Binning”, “Encoding”, “Scaling”, “Target”, “Sample Weight”).
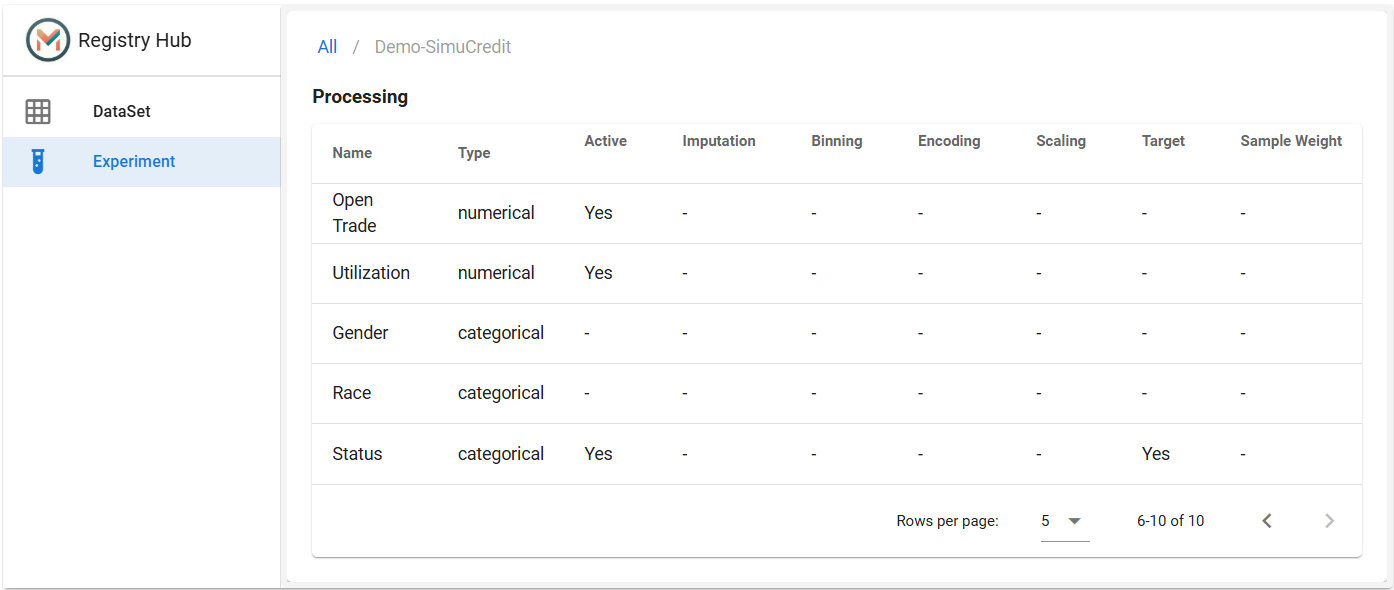
View Models: Click in the Model column to view trained models (e.g., “XGBoost”).
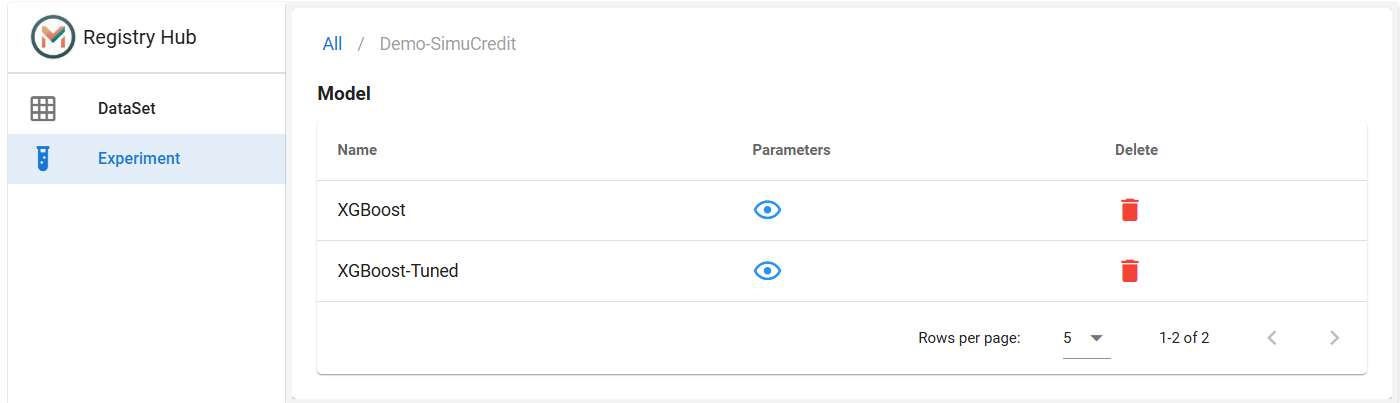
Hyperparameters: Click
in the Parameter column to view parameters like max_depth, n_estimators, and random_state.
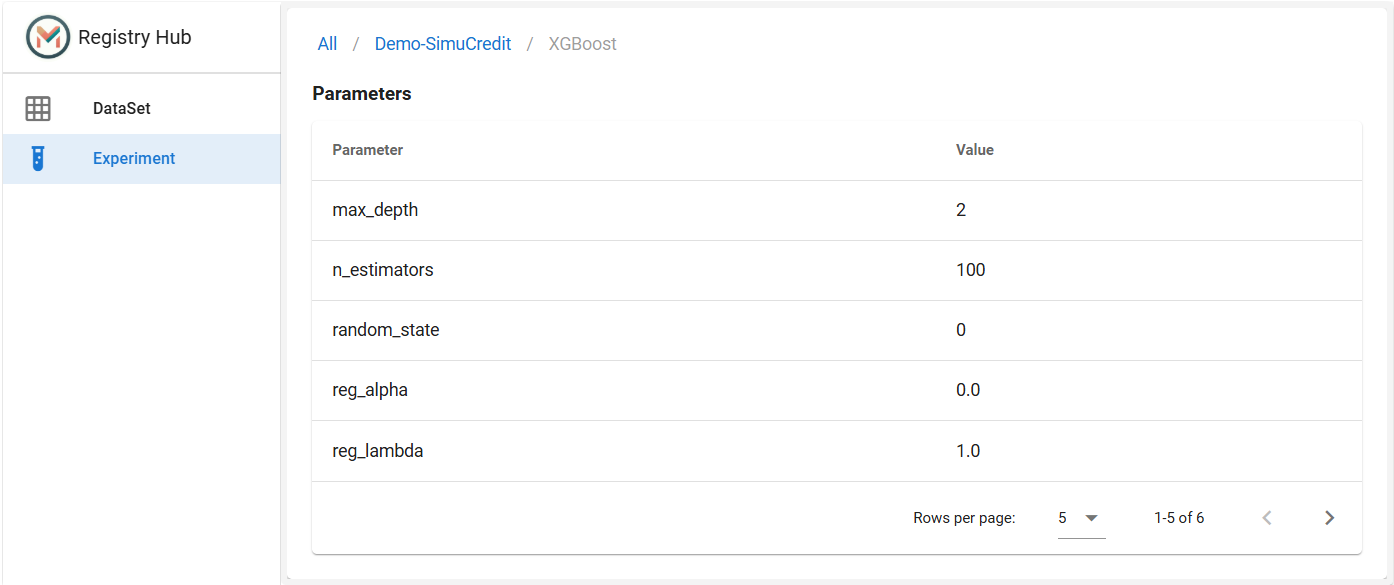
View TestSuites: Click in the Testsuite column to explore test results (e.g., “Categorical-Result”, “Numeric-Result”).
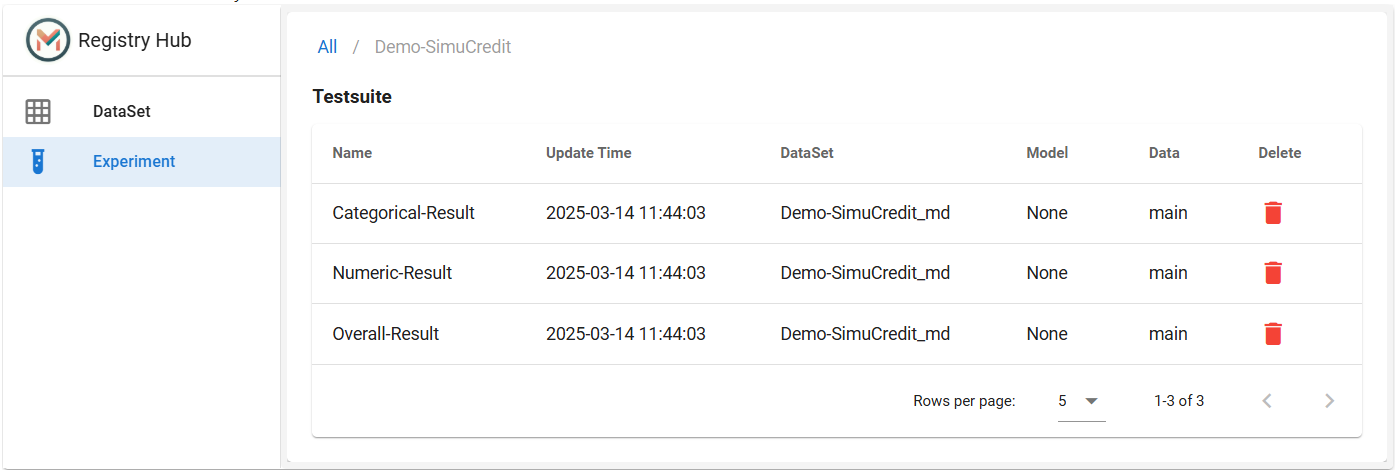
Delete Experiment: Click to remove an experiment and all associated data.
Warning
Deletion permanently removes all experiment data, including models and test results.
The Registry Hub panel provides a centralized location for managing datasets, experiments, models, and test suites. It simplifies the tracking and management of ML processes, enabling users to efficiently monitor and manage their projects.