Model Explainability#
The Model Explainability panel provides insights into how a machine learning model makes predictions using global (whole-model) and local (per-sample) explanations.
Initialize the Panel#
To create and initialize the Model Explainability panel, use:
# Load the Experiment and test explainability
from modeva import Experiment
exp = Experiment(name='Demo-SimuCredit')
exp.test_explainability()
Workflow#
Step 1: Load and Select Dataset#
Select a Dataset: The dataset from the dropdown for processing is automatically selected based on the processed dataset of the experiment (e.g.,
Demo-SimuCredit_md
).Set the Data Selection: Choose a data split (e.g.,
test
).Set Select Model: Pick a registered model from the dropdown (e.g.,
XGBoost
).
Step 2: Global Explanations#
Switch to the Global tab to analyze feature importance and effects across the dataset.
Configure Explanation Method:
Inherent Interpretability: Uses model-specific insights (Only for interpretable models).
Post-hoc Explainability: Uses PFI (Permutation Feature Importance) and PDP (Partial Dependence Plot) for model-agnostic analysis.
Choose Feature: - Select a feature by clicking on the bar in the feature importance plot to view its effect on the model prediction.
Step 3: Local Explanations#
Switch to the Local tab to analyze individual predictions.
Configure Explanation Method:
Inherent Interpretability: View local effects for interpretable models.
Post-hoc Explainability: Use LIME (Local Interpretable Model-agnostic Explanations) and SHAP (Shapley Additive Explanations) for black-box models.
Select Sample Index: - Enter a sample index (e.g., 42) to analyze.
Step 4: Saving Results#
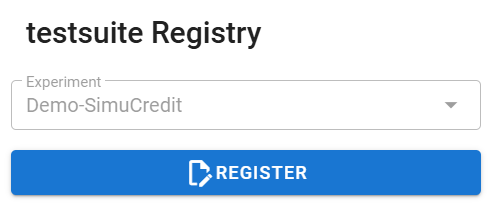
This panel bridges the gap between model complexity and interpretability. Use it to validate feature logic, debug predictions, and meet regulatory requirements. For more information, refer to the Model Explainability and Interpretable Models.