Model Training#
The Model Training panel allows users to train, evaluate, and register machine learning models efficiently. The panel also provides a leaderboard to compare model performance and register the best-performing models.
Workflow#
# Load the Experiment and train a model
from modeva import Experiment
exp = Experiment(name='Demo-SimuCredit')
exp.model_train()
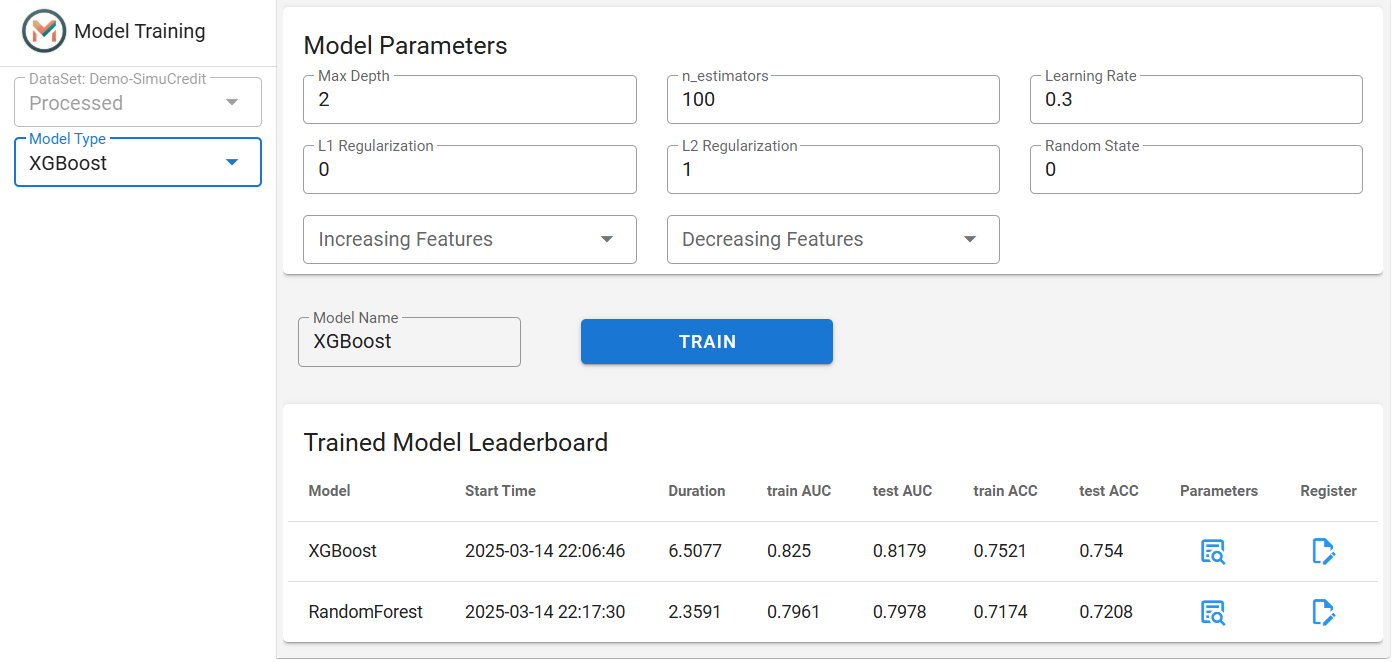
Step 1: Dataset & Model Selection#
Select a Dataset: The dataset from the dropdown for processing is automatically selected based on the processed dataset of the experiment (e.g.,
Demo-SimuCredit_md
).Choose Model Type: Open the Model Type dropdown to select an algorithm (e.g.,
XGBoost
,LightGBM
,CatBoost
…). The model object is auto-initialized based on the dataset task type (Regression/Classification).
Step 2: Train a Model#
Name Your Model: Enter a unique name in the Model Name field (e.g.,
XGBoost
).Adjust Hyperparameters: The hyperparameters settings are auto-initialized based on the selected model type. Users can modify these settings, such as
learning_rate
,max_depth
and other hyperparameters to optimize model performance.Start Training: Click the Train button to begin training.
Monitor Progress: A progress bar indicates training status. Once completed, results appear in the Trained Model Leaderboard.
Step 3: Evaluate and Compare Models#
Trained Models Leaderboard:
Displays model performance metrics such as
AUC
andACC
for classification tasks, andMSE
andMAE
for regression tasks.Key columns also include
Model name
,Start Time
andDuration
.
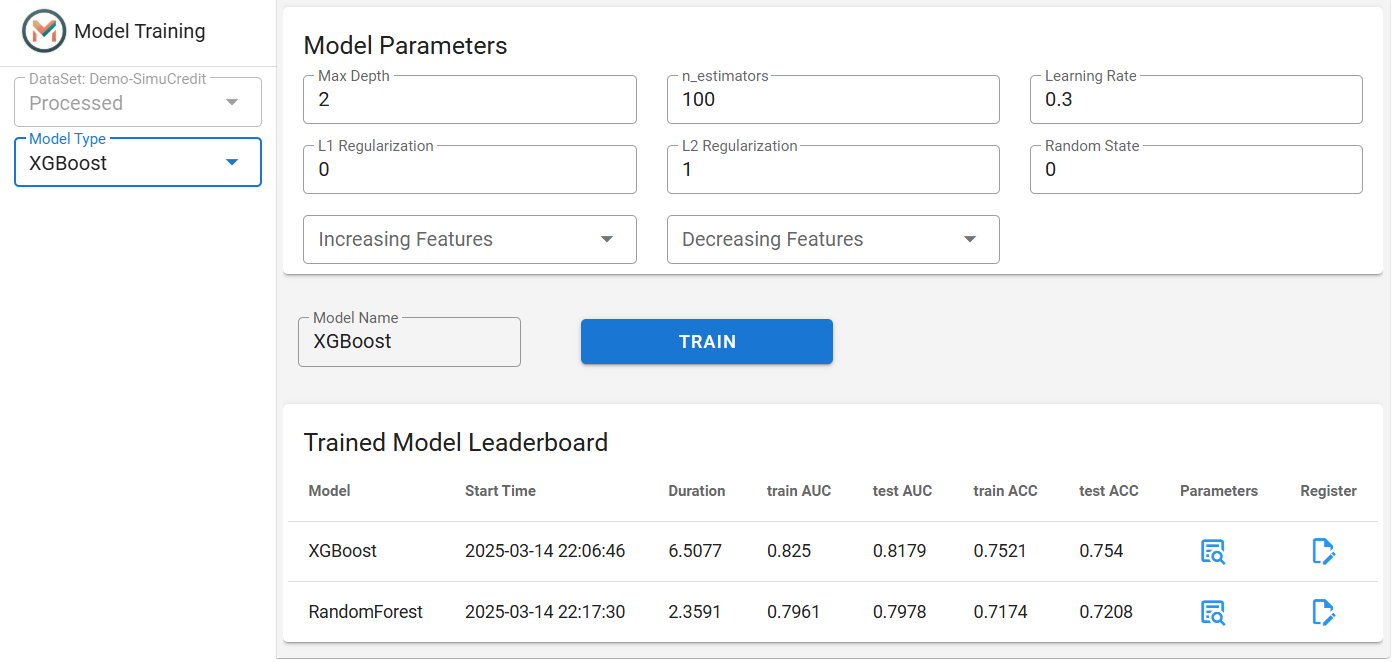
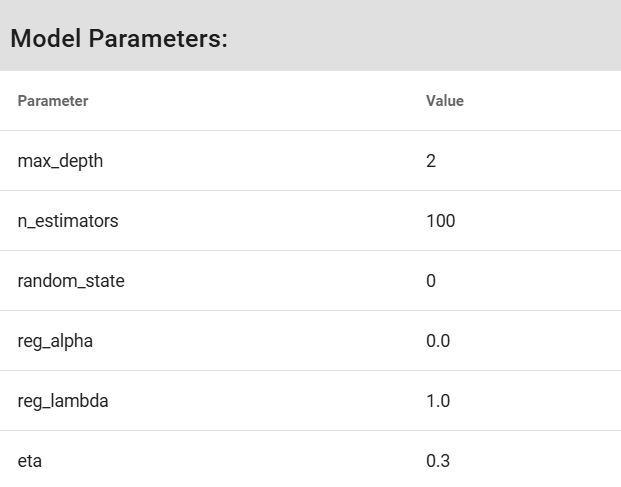
Step 4: Register a Model#
- Save a Trained Model:
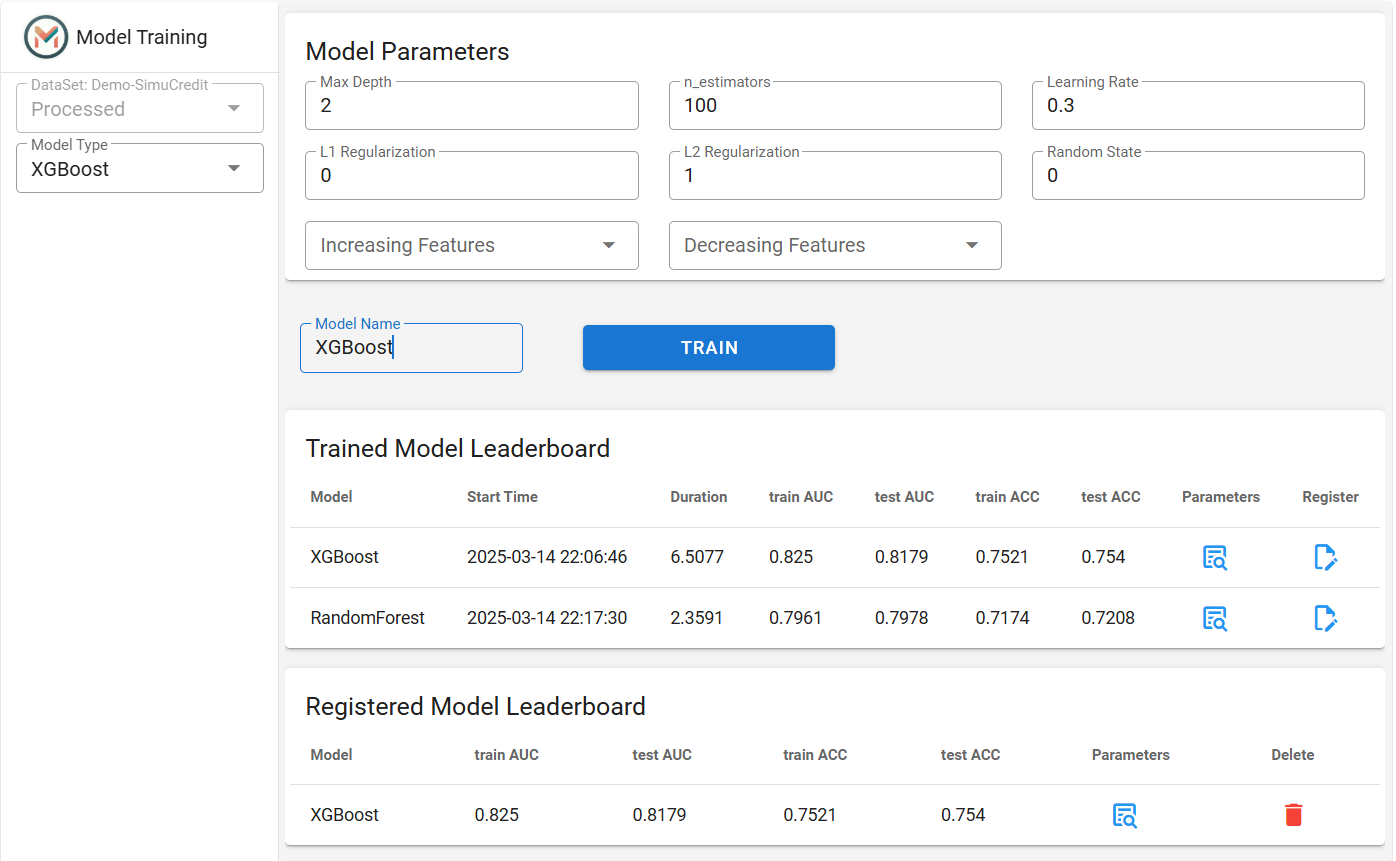
This panel streamlines model experimentation and deployment. Users can train, evaluate, and register models with minimal effort, enabling rapid prototyping and deployment. For advanced users, the panel offers extensive customization options to fine-tune model performance. For more information, refer to the Model Zoo and Leaderboard.