Model Tuning#
The Model Tuning panel allows users to optimize machine learning models through hyperparameter tuning and register the best-performing configurations.
Initialize the Panel#
To create and initialize the Model Tuning panel, use:
# Load the Experiment and tune a model
from modeva import Experiment
exp = Experiment(name='Demo-SimuCredit')
exp.model_tune()
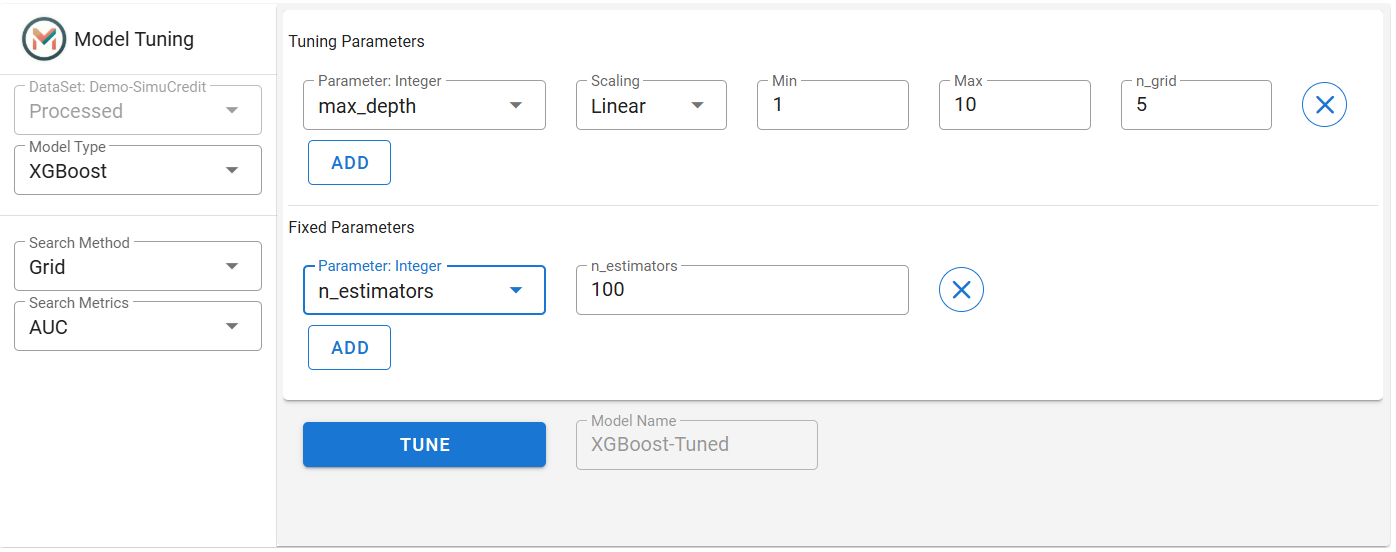
Workflow#
Step 1: Select Dataset, Model, and Search Method#
Select a Dataset: The dataset from the dropdown for processing is automatically selected based on the processed dataset of the experiment (e.g.,
Demo-SimuCredit_md
).Choose Model Type: Select an algorithm from the Model Type dropdown (e.g.,
XGBoost
,LightGBM
).Select Search Method:
Grid: Exhaustive search over predefined parameter combinations.
Random: Randomized sampling of parameter combinations (specify iterations).
Choose Metrics: Select evaluation metrics (support multiple metrics) for tuning.
For regression tasks, use metrics like
MSE
,MAE
, andR2
.For classification tasks, available metrics include
AUC
,ACC
,F1
,LogLoss
, andBrier
.
Step 2: Configure Tuning Parameters#
Add Tuning Parameters
Click ADD under Tuning Parameters to define the parameters search space.
For numerical parameters: Set
min
,max
, grid size, and scaling (options:Linear
,Logarithmic
,Exponential
).For categorical parameters: Select available values (e.g.,
[0.1, 0.2, 0.3]
).
Add Fixed Parameters
Click ADD under Fixed Parameters to lock specific hyperparameters (e.g.,
n_estimators=100
).
Step 3: Run Tuning#
Name Your Model: Enter a name in the Model Name field (e.g.,
XGB-Tuned
).Start Tuning: Click the Tune button. A progress bar will indicate execution status.
View Results:
Parallel Coordinate Plot visualizes parameter combinations and their performance. Lines represent parameter combinations; colors indicate performance. Hover to see metric values and parameter details.
Tuning Leaderboard ranks models by the selected metrics. Key columns include
Model Name
,Start Time
,Duration
, and evaluation metrics.
Step 4: Register the Best Model#
Register a Model: In the leaderboard, click the button next to a model row.
Troubleshooting#
Invalid Parameters: Ensure
min
≤max
for numerical ranges. Avoid duplicate parameters in tuning/fixed sections.Slow Tuning: Reduce grid size for Grid Search or iterations for Random Search.
This panel streamlines hyperparameter optimization for robust model performance. Users can compare and register the best configurations for future use. For more information, refer to the Model Tuning.