Fairness#
Group fairness refers to ensuring that models make predictions equitably across different demographic or protected groups defined by sensitive attributes such as gender, race, or age. Group fairness is critical for preventing systematic discrimination and building models that are ethical, trustworthy, and compliant with legal standards.
Definitions of Group Fairness#
There are several formalized definitions of group fairness, each designed for specific contexts. Selecting the appropriate definition depends on the application and fairness goals.
Demographic Parity (Statistical Parity):
Ensures that the rate of positive outcomes is the same across all groups.
Formula:
\[P(\hat{y} = 1 | A = a) = P(\hat{y} = 1 | A = b) \quad \forall a, b\]Example: In hiring models, ensure the selection rate is equal for different genders.
Limitations:
Does not account for differences in the actual distribution or base rates of outcomes across groups.
Equal Opportunity:
Ensures that the true positive rate is the same for all groups.
Formula:
\[P(\hat{y} = 1 | y = 1, A = a) = P(\hat{y} = 1 | y = 1, A = b) \quad \forall a, b\]Example: In credit scoring, ensure that those who repay loans are approved equally across groups.
Strength:
Focuses on fairness for the positive class, especially important in high-stakes decisions.
Equalized Odds:
Requires both the true positive rate (TPR) and the false positive rate (FPR) to be equal across groups.
Formula:
\[P(\hat{y} = 1 | y = c, A = a) = P(\hat{y} = 1 | y = c, A = b) \quad \forall a, b, c \in \{0, 1\}\]Example: In healthcare diagnostics, ensure both detection rates and misdiagnoses are balanced across groups.
Strength:
Provides a more comprehensive fairness guarantee by addressing both types of errors.
Conditional Parity:
Ensures fairness under specific conditions, such as controlling for legitimate risk factors.
Example: Insurance pricing models may enforce parity within risk-adjusted subgroups.
Metrics for Group Fairness#
To measure group fairness, several metrics evaluate the extent to which fairness criteria are satisfied.
Disparate Impact (DI):
Measures the ratio of positive outcome rates between unprivileged and privileged groups.
Formula:
\[\text{Disparate Impact} = \frac{P(\hat{y} = 1 | A = \text{unprivileged})}{P(\hat{y} = 1 | A = \text{privileged})}\]A DI ratio close to 1 indicates fairness.
Threshold: A ratio between 0.8 and 1.25 is often used as a fairness benchmark (80% rule).
Statistical Parity Difference:
Measures the difference in positive outcome rates between groups.
Formula:
\[\text{Statistical Parity Difference} = P(\hat{y} = 1 | A = a) - P(\hat{y} = 1 | A = b)\]
Equality of Opportunity Difference:
Measures the difference in true positive rates between groups.
Formula:
\[\text{Equality of Opportunity Difference} = P(\hat{y} = 1 | y = 1, A = a) - P(\hat{y} = 1 | y = 1, A = b)\]
Equalized Odds Difference:
Measures the largest difference in true positive and false positive rates between groups.
Formula:
\[\begin{split}\text{Equalized Odds Difference} = \max \left( |P(\hat{y} = 1 | y = 1, A = a) - P(\hat{y} = 1 | y = 1, A = b)|, \\ |P(\hat{y} = 1 | y = 0, A = a) - P(\hat{y} = 1 | y = 0, A = b)| \right)\end{split}\]
Calibration Across Groups:
Ensures predicted probabilities are well-calibrated for each group.
Formula:
\[P(y = 1 | \hat{p}, A = a) = P(y = 1 | \hat{p}, A = b) \quad \forall \hat{p}\]
Adverse Impact Ratio (AIR) for Disparate Impact#
Adverse Impact Ratio (AIR) is a key metric used to measure disparate impact in machine learning models. It quantifies the disparity in favorable outcomes (e.g., loan approval, hiring) between unprivileged and privileged groups, helping to identify potential unfairness in decision-making systems.
The formula for AIR is:
Where:
\(P(\hat{y} = 1 | A = \text{unprivileged})\): Probability of a favorable outcome for the unprivileged group.
\(P(\hat{y} = 1 | A = \text{privileged})\): Probability of a favorable outcome for the privileged group.
\(A\): Sensitive attribute (e.g., gender, race).
Interpretation of AIR
AIR = 1: Indicates perfect parity between the unprivileged and privileged groups (no disparate impact).
AIR < 1: Suggests potential disparate impact against the unprivileged group (e.g., lower approval rates).
AIR > 1: Indicates potential favorable bias toward the unprivileged group.
80% Rule (Fairness Threshold)
The 80% rule, commonly used in fairness evaluations, establishes a threshold for AIR:
AIR ≥ 0.8 (80%): Generally considered fair under the rule.
AIR < 0.8: May indicate significant disparity, requiring further investigation and potential remediation.
Strengths and Limitations of AIR
Strengths:
Easy to compute and interpret.
Provides a simple measure of disparity.
Aligns with legal and regulatory standards (e.g., U.S. Equal Employment Opportunity Commission (EEOC) guidelines).
Limitations:
Does not account for differences in base rates across groups, which can lead to misleading conclusions.
Focuses only on positive outcomes and does not assess fairness for negative outcomes (e.g., false positives).
May conflict with other fairness metrics such as Equalized Odds or Equal Opportunity.
AIR is an essential metric for assessing group fairness and detecting disparate impact in machine learning models. By comparing favorable outcome rates between unprivileged and privileged groups, AIR helps practitioners evaluate fairness and align with ethical and legal standards. However, AIR should be interpreted alongside other fairness metrics for a comprehensive understanding of model behavior.
3. Examples and Applications
Hiring Models:
Ensure selection rates for different genders or ethnicities are similar (statistical parity).
Credit Scoring:
Evaluate true positive rates (loan approvals for repaid loans) and false positive rates (loan approvals for defaults) for different demographic groups.
Healthcare Diagnostics:
Assess the balance of true and false positive rates across groups, such as ensuring fair detection rates for diseases across genders.
4. Challenges in Group Fairness
Conflicting Fairness Metrics:
Achieving one fairness criterion may violate another. For example, satisfying equalized odds may conflict with demographic parity when base rates differ.
Tradeoffs with Accuracy:
Enforcing fairness constraints may lead to reduced overall accuracy, especially when group distributions differ.
Data Limitations:
Insufficient representation of minority groups can lead to unreliable fairness assessments.
Cultural and Contextual Factors:
Fairness definitions may vary by application and societal norms.
Group fairness measurement is a critical component of building equitable machine learning models. By leveraging fairness metrics such as disparate impact, statistical parity difference, and equalized odds, practitioners can identify and address biases to ensure that models operate fairly across diverse demographic groups. While challenges like metric conflicts and accuracy tradeoffs exist, adopting fairness-aware practices helps create models that are both effective and socially responsible.
Fairness Metrics in MoDeVa#
MoDeVa supports various fairness metrics, as shown below. In specific, we use the subscripts \(p\) and \(r\) to denote the protected and reference groups, respectively.
AIR: Adverse impact ratio.
Precision: Positive predictive value disparity ratio.
Recall: True positive rate disparity ratio.
SMD: Standardized mean difference between protected and reference groups. (Only used for regression tasks)
Fairness Evaluation in MoDeVa#
Data Setup
from modeva import DataSet
# Create dataset object holder
ds = DataSet()
# Loading MoDeVa pre-loaded dataset "Bikesharing"
ds = DataSet(name="TaiwanCredit")
ds.load("TaiwanCredit")
# Encode categorical data into ordinal
ds.encode_categorical(method="ordinal")
# Execute data pre-processing
ds.preprocess()
# Set target variable
ds.set_target("FlagDefault")
# Set the following variables as inactive (not used for modeling)
ds.set_inactive_features(["SEX", "MARRIAGE", "AGE"])
# Randomly split training and testing set
ds.set_random_split()
Model Setup
# Classification tasks using lightGBM and xgboost
from modeva.models import MoLGBMClassifier, MoXGBClassifier
# for lightGBM
model_lgbm = MoLGBMClassifier(name = "LGBM_model", max_depth=2, n_estimators=100)
# for xgboost
model_xgb = MoXGBClassifier(name = "XGB_model", max_depth=2, n_estimators=100)
Model Training
# train model with input: ds.train_x and target: ds.train_y
model_lgbm.fit(ds.train_x, ds.train_y)
model_xgb.fit(ds.train_x, ds.train_y)
Reporting and Diagnostic Setup
# Create a testsuite that bundles dataset and model
from modeva import TestSuite
ts = TestSuite(ds, model_lgbm) # store bundle of dataset and model in fs
# Evaluate performance and summarize into table
results = ts.diagnose_accuracy_table(train_dataset="train", test_dataset="test")
results.table
Setting “protected data” status for fairness evaluation
#set protected data
ds.set_protected_data(ds.raw_data[["SEX", "MARRIAGE", "AGE"]])
# Create configuration to define protected and reference groups
group_config = {
"Gender": {"feature": "SEX", "protected": 1.0, "reference": 2.0},
"MARRIAGE": {"feature": "MARRIAGE", "protected": 2.0, "reference": 1.0},
"AGE": {"feature": "AGE", "protected": {"lower": 60, "lower_inclusive": True},
"reference": {"upper": 60, "upper_inclusive": False}}
}
# Evaluate model fairness
results = ts.diagnose_fairness(group_config=group_config,
favorable_label=1,
metric="AIR",
threshold=0.8)
results.plot()
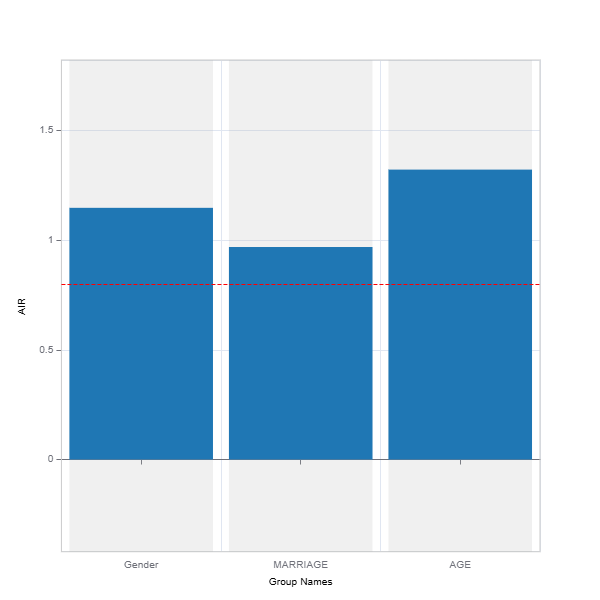
For the full list of arguments of the API see TestSuite.diagnose_fairness.
Slicing for Fairness Diagnostics#
1. Univariate Slicing
results = ts.diagnose_slicing_fairness(features="PAY_1",
group_config=group_config,
dataset="train",
metric="AIR")
results.plot()
For the full list of arguments of the API see TestSuite.diagnose_slicing_fairness.
2. Bivariate Slicing
results = ts.diagnose_slicing_fairness(features=("PAY_1", "BILL_AMT1"),
group_config=group_config,
dataset="train",
metric="AIR",
threshold=0.9)
results.plot("MARRIAGE")
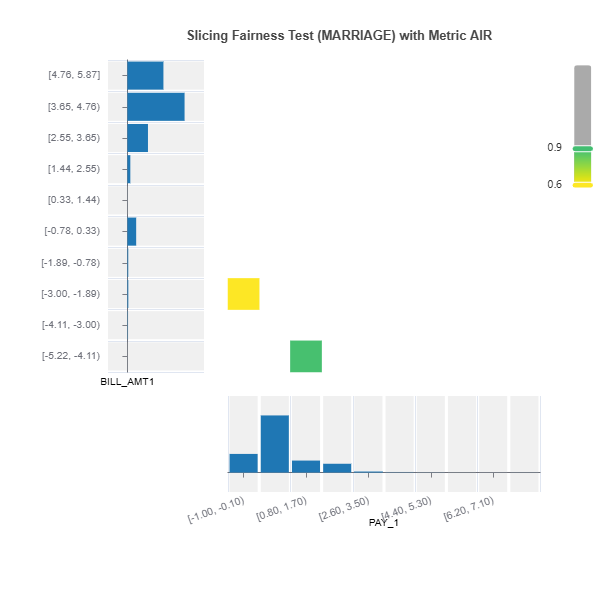
3. Evaluation for All Features
# Create tuple for feature list in ds.features_names
feature_names = tuple((x,) for x in ds.feature_names)
results = ts.diagnose_slicing_fairness(features=feature_names,
group_config=group_config,
dataset="train",
metric="AIR",
method="auto-xgb1", bins=5)
results.table["MARRIAGE"]
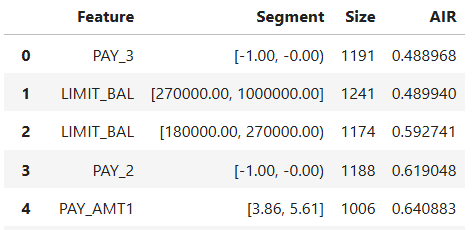
Fairness Comparison#
Fairness of several models can be compared as follows:
tsc = TestSuite(ds, models=[model_lgbm, model_xgb])
results = tsc.compare_fairness(group_config=group_config, metric="AIR", threshold=0.8)
results.plot()
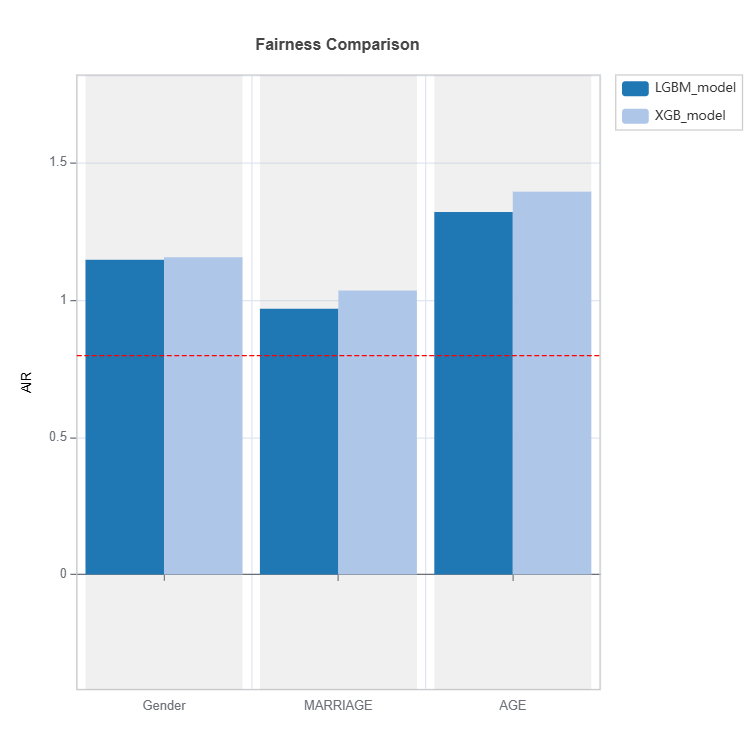
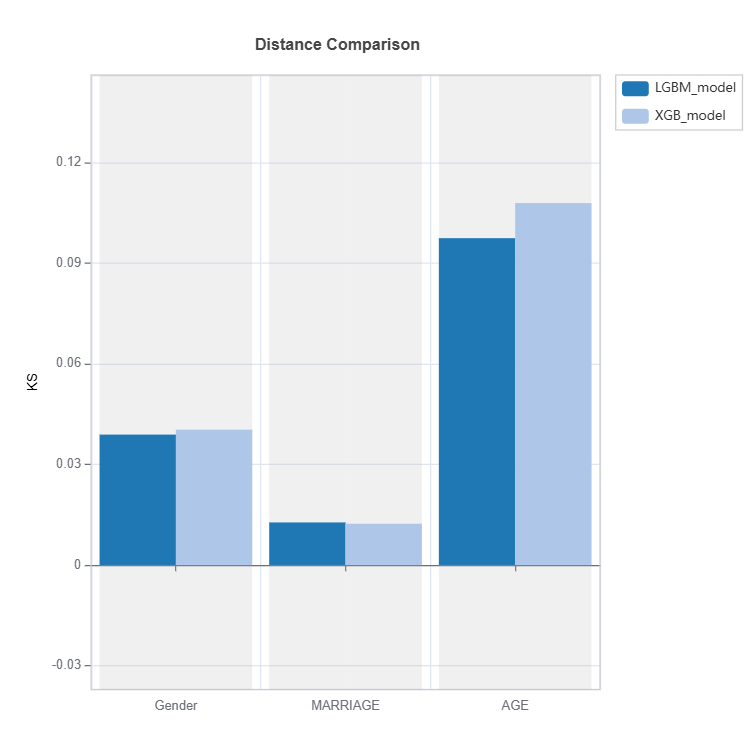
For the full list of arguments of the API see TestSuite.compare_fairness.
result = tsc.compare_slicing_fairness(features="BILL_AMT1",
group_config=group_config,
favorable_label=1,
dataset="train",
metric="AIR")
print(result.table["XGB_model"]["MARRIAGE"])
print(result.table["LGBM_model"]["MARRIAGE"])
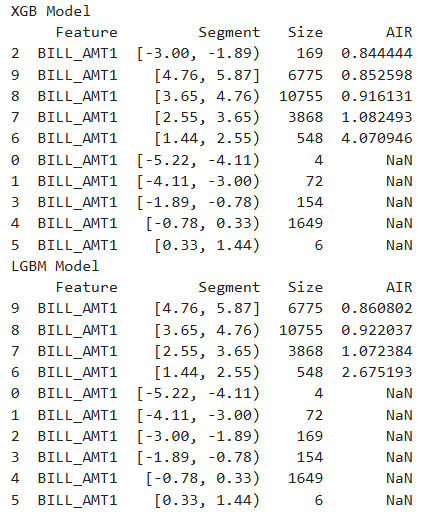
“NaN” values occur for bins where threre are only one class (protected or reference)
For the full list of arguments of the API see TestSuite.compare_slicing_fairness.
Fairness Mitigation#
Fairness mitigation in machine learning aims to reduce bias in model predictions to ensure equitable outcomes across demographic groups. Two effective debiasing methods include threshold adjustment and feature binning. Both methods involve tradeoffs between performance and fairness metrics, such as the Adverse Impact Ratio (AIR).
Threshold Adjustment#
Threshold adjustment changes the decision threshold of a model to balance performance and fairness across groups. This method directly modifies the conditions under which a favorable outcome (e.g., loan approval) is granted.
Impact on AIR:
Lowering the threshold for the unprivileged group increases the probability of favorable outcomes for that group, improving AIR.
Raising the threshold for the privileged group can reduce disparities but may decrease overall model performance.
Tradeoffs:
Fairness: Threshold adjustment can significantly improve fairness metrics like AIR and statistical parity.
Performance: Introducing group-specific thresholds may lead to reduced overall accuracy or increased false positives/negatives.
Example in MoDeVa:
result = ts.diagnose_mitigate_unfair_thresholding(group_config=group_config,
favorable_label=1,
dataset="train",
metric="AIR",
performance_metric="ACC",
proba_cutoff=(0.1, 0.2, 0.3, 0.4, 0.5, 0.6, 0.7, 0.8, 0.9))
result.table
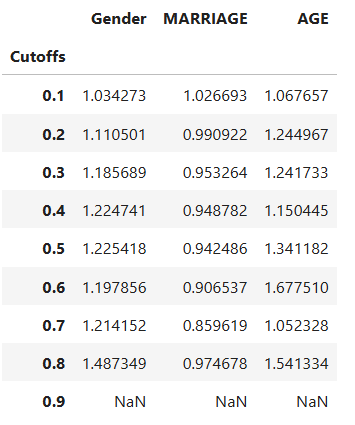
Feature Binning#
Feature binning reduces the granularity of input features by grouping them into fewer bins. This simplification can reduce disparities caused by overly precise or biased feature representations.
How It Works:
Granular Features:
Features like income or credit score may have many fine-grained levels, leading to group disparities.
Reduced Binning:
Aggregate feature values into broader bins (e.g., low, medium, high) to smooth differences across groups.
Impact on AIR:
Coarser bins can reduce disparities by limiting the model’s ability to overfit group-specific nuances.
Broader bins may increase statistical parity but may lose some predictive performance.
Tradeoffs:
Fairness: Reducing bins can improve fairness metrics such as AIR by creating more uniform feature distributions across groups.
Performance: Coarser binning may lead to a loss of model precision, reducing accuracy or predictive power.
Example in MoDeVa:
result = ts.diagnose_mitigate_unfair_binning(group_config=group_config,
favorable_label=1,
dataset="train",
metric="AIR",
performance_metric = "AUC",
binning_method = "quantile",
bins = 5)
result.plot()
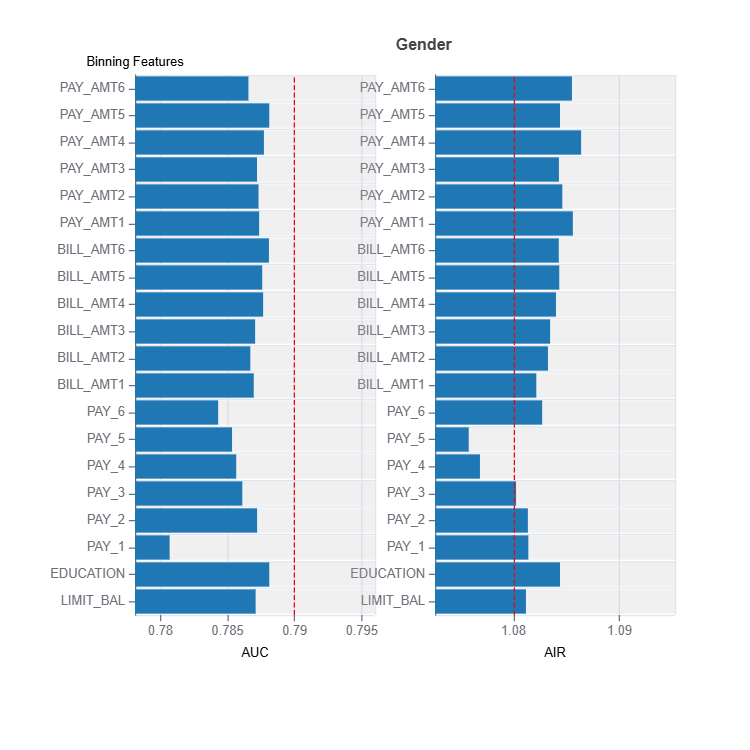
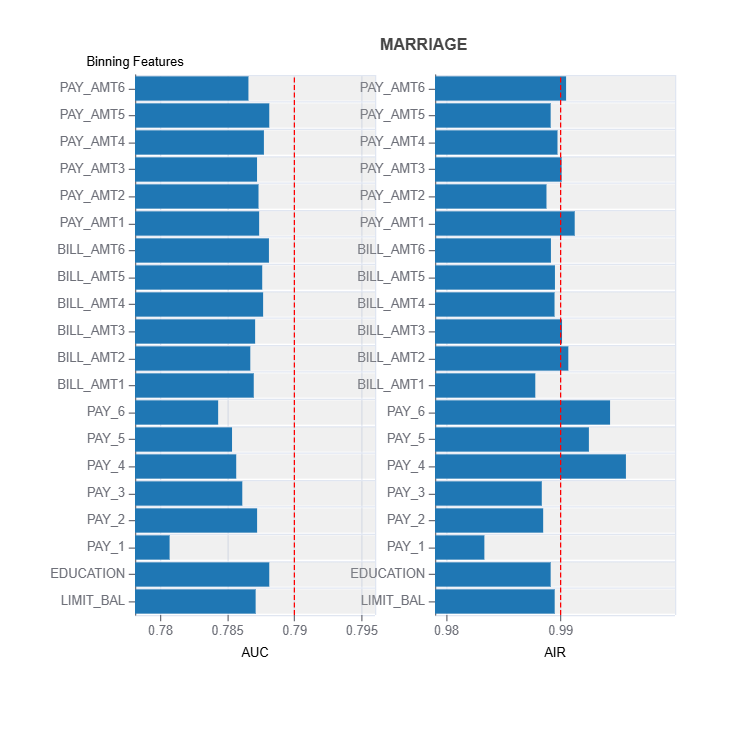
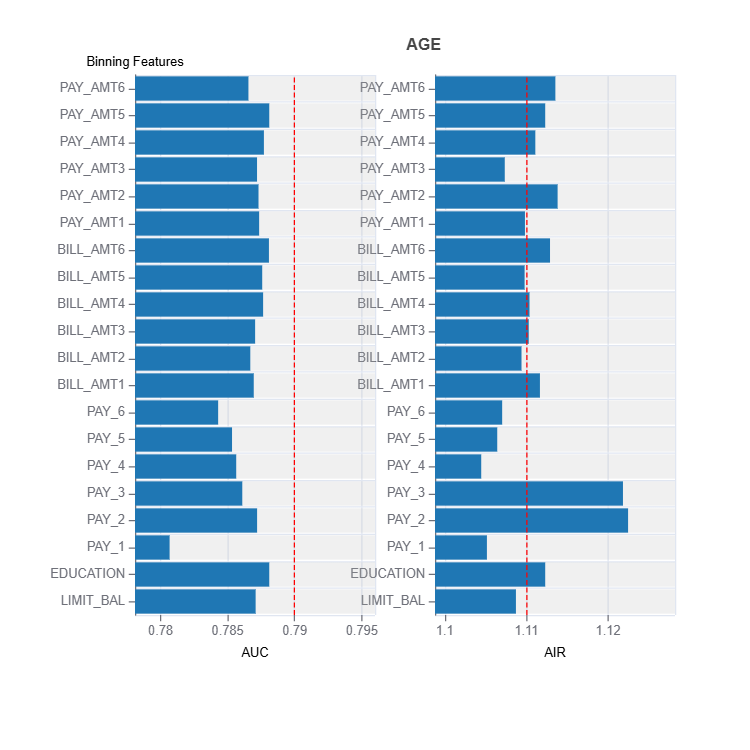
Tradeoffs Between Performance and Fairness#
When applying debiasing techniques like threshold adjustment or feature binning, there are inherent tradeoffs between performance and fairness:
Performance:
Adjusting thresholds or reducing feature granularity can lead to decreased accuracy, precision, or recall.
Loss of fine-grained information may result in reduced model efficiency for certain subgroups.
Fairness:
Debiasing methods can improve metrics like AIR, statistical parity, or equalized odds, ensuring more equitable outcomes.
Fairness improvements may come at the cost of overall predictive performance.
Combined Application of Threshold Adjustment and Feature Binning#
Threshold adjustment and feature binning can be used together to address fairness concerns while minimizing performance loss:
Threshold Adjustment: Modify decision thresholds to balance group outcomes.
Feature Binning: Simplify input features to reduce disparities in feature-driven predictions.
By carefully monitoring fairness metrics (e.g., AIR) and performance metrics (e.g., accuracy, AUC), we can balance these competing objectives.
Debiasing methods like threshold adjustment and feature binning are effective techniques for fairness mitigation. While they involve tradeoffs between performance and fairness, these techniques help ensure more equitable outcomes across groups, aligning machine learning models with ethical and regulatory standards.
Examples#
An example codes of this section can be found in the following link.