Reliability#
Reliability in predictive models refers to their ability to produce consistent and trustworthy outputs. Model reliability measures the confidence in a model’s predictions by identifying areas of high uncertainty and ensuring consistency over time. This is particularly important in high-stakes domains like banking, where unreliable predictions can lead to significant risks or losses.
Output uncertainty, or lack of confidence in predictions, arises from factors such as noisy data, sparse samples, or inherent model limitations.
Investigating and Improving Model Reliability
In MoDeVa, investigating and improving model reliability involves the following steps:
Uncertainty Quantification: Conformal Prediction (CP) is used to generate prediction intervals, providing a range within which the true value is likely to fall with a specified confidence level.
Identification of Less Reliable Regions: Model weakness identification involves pinpointing areas in the data where the model performs poorly or lacks confidence. In MoDeVa, this is achieved by examining two key factors: (1) High uncertainty regions and (2) Outside Conformal Prediction coverage.
Determine Impactful Variables: Identify key variables whose contribute to output uncertainty.
Enhance the Model: Address the identified issues by refining the model, such as through further analysis, introducing segment-specific models, or other tailored adjustments.
Conformal Prediction#
Conformal prediction provides a model-agnostic framework for constructing prediction intervals with guaranteed coverage under minimal assumptions. The key idea is to quantify how different a new test point is from the training data using a nonconformity score.
The framework offers finite-sample, distribution-free coverage guarantees requiring only that the data are exchangeable. For a desired miscoverage rate α, conformal prediction produces prediction intervals that contain the true value with probability 1-α.
Full Conformal Prediction#
Full conformal prediction requires refitting the model for each test point, making it computationally expensive.
Mathematical Formulation:
For a dataset \({(X_,Y_),...,(X_n,Y_n)}\) and test point \(X_{n+1}\):
where:
\(\pi_y\) is the p-value for candidate y
For each \(y\), refit model on \({(X_,Y_),...,(X_n,Y_n),(X_{n+1},y)}\)
Compute nonconformity scores and compare to calibration set
To reduce the computational burden, an approximate approach is used at the expense of reduction of coverage guarantee.
Split Conformal Prediction#
Split conformal reduces computational cost by splitting data into proper training and calibration sets.
Algorithm:
Split data into training \(D_{\text{train}}\) and calibration \(D_{\text{cal}}\) sets
Train model on \(D_{\text{train}}\)
Compute nonconformity scores on \(D_{\text{cal}}\)
Use empirical quantiles of scores to construct prediction intervals
Binary Classification
For binary classification, nonconformity scores typically use predicted probabilities.
Nonconformity Score:#
- where:
\(\hat{p}_i\) is predicted probability for class 1
Lower scores indicate better conformity with training data
Conformalized Residual Quantile Regression (CRQR)
CRQR applies quantile regression to the residuals to estimate prediction intervals.
Algorithm
1. Base Model Fitting:
First, fit a base model \(f(X)\) to estimate conditional mean:
Calculate residuals:
2. Residual Quantile Regression:
Train quantile regression on residuals for desired quantiles \(\tau_1\), \(\tau_2\):
3. Conformalization:
Compute nonconformity scores on calibration set:
4. Prediction Intervals:
For new point \(X\), construct interval:
where:
\(\hat{f}(X)\) is base model prediction
\(\hat{q}_{τ}(X)\) are predicted residual quantiles
\(Q_{1-α}(s)\) is (1-α) quantile of calibration scores
Advantages and Considerations
Distribution-free coverage guarantees
Works with any base predictor
Split conformal trades statistical efficiency for computational efficiency
Residual approach can capture heteroscedasticity
Can be extended to handle covariate shift
Reliability Analysis in MoDeVa#
Data Setup
from modeva import DataSet
# Create dataset object holder
ds = DataSet()
# Loading MoDeVa pre-loaded dataset "Bikesharing"
ds.load(name="BikeSharing") # Changed dataset name
## Preprocess the data
ds.scale_numerical(features=("cnt",), method="log1p") # Log transfomed target
ds.set_feature_type(feature="hr", feature_type="categorical") # set to categorical feature
ds.set_feature_type(feature="mnth", feature_type="categorical")
ds.scale_numerical(features=ds.feature_names_numerical, method="standardize") # standardized numerical features
ds.set_inactive_features(features=("yr", "season", "temp")) # deactivate some features
ds.preprocess()
## Split data into training and testing sets randomly
ds.set_random_split()
Model Setup
# Regression tasks using lightGBM and xgboost
from modeva.models import MoLGBMRegressor, MoXGBRegressor
# for lightGBM
model_lgbm = MoLGBMRegressor(name = "LGBM_model", max_depth=2, n_estimators=100)
# for xgboost
model_xgb = MoXGBRegressor(name = "XGB_model", max_depth=2, n_estimators=100)
Model Training
# train model with input: ds.train_x and target: ds.train_y
model_lgbm.fit(ds.train_x, ds.train_y)
model_xgb.fit(ds.train_x, ds.train_y)
Reporting and Diagnostic Setup
# Create a testsuite that bundles dataset and model
from modeva import TestSuite
ts = TestSuite(ds, model_lgbm) # store bundle of dataset and model in fs
Reliability Assessment:#
In the example of regression task below, split conformal prediction is used.
Expected coverage of prediction intervals, \(\alpha\) = 0.1
Test dataset is split into:
50% of data is used to train Quantile regression using LightGBM with tree depth 5 for the residuals and calibration
50% of data and testing
# reliability assessment using Split Conformal Prediction
results = ts.diagnose_reliability(
train_dataset="test", # train dataset
test_dataset="test",
test_size=0.5,
alpha=0.1,
max_depth=5,
random_state=0)
results.table
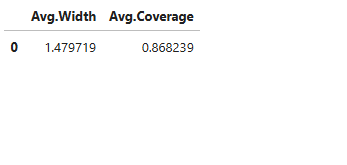
The table shows the average width of prediction interval and the coverage.
For the full list of arguments of the API see TestSuite.diagnose_reliability.
Identification of Reliability Issue and Impactful Variables#
There are several ways to identify reliability issues (finding region in the input space with high uncertainty): * Apply slicing technique to identify variables and their intervals with less reliable outputs * Fit inherently interpretable model (e.g., xgboost depth-2) with prediction reliability (or in our out coverage) as target and plot the effects (main or interaction) as well as variable importance with respected to reliability measure. * Select sub-samples with less reliable outputs and visualize and compare the distributions between unreliable vs. the rest. Distribution difference can be quantified using measure such as PSI.
Less reliable outputs can be identified by analyzing two key aspects of conformal prediction outputs:
High Uncertainty
Out-of-Coverage Instances
For Regression:
Measure prediction interval width:
Flag points where:
where:
\(\beta\) is threshold (e.g., 0.9 for top 10% widest intervals)
\(Q_1₋\beta(W)\) is the \((1-\beta)\) quantile of interval widths
For Binary Classification:
Flag instances where:
where:
\(|C_{n,\alpha}(X)|\) = 0: Empty prediction set (high uncertainty)
\(|C_{n,\alpha}(X)|\) = 2: Both classes predicted (high uncertainty)
1. Coverage Violation Detection:
For true value \(y\) and prediction set \(C_{n,\alpha}(X)\):
2. Region Characterization:
Analyze feature distributions where:
- where:
\(R\) is a region in feature space
\(\alpha\) is nominal miscoverage rate
Common patterns indicating model weakness:
High uncertainty + good coverage: Model is honest about uncertainty
High uncertainty + poor coverage: Model may be misspecified
Low uncertainty + poor coverage: Model is overconfident
Reliability Diagnostics in MoDeVa#
To understand the region with higher uncertainty can be analyzed as follows:
data_results = ds.data_drift_test(
**results.value["data_info"],
distance_metric="PSI",
psi_method="uniform",
psi_bins=10)
The results of distribution between less reliable region vs. overal data from TestSuite.diagnose_reliability is evaluated using DataSet.data_drift_test
Percent of worst sample = 0.1 (10%) is selected for least reliable
Metrics: PSI with 10 uniform binning is used for calculation.
data_results.plot() displays the following results:
Summary of distribution differences for all the features ranked by PSI
Marginal density comparison of distribution shift
Marginal histogram comparison of distribution shift
Summary of variable ranked according to their PSI (distribution difference)
data_results.plot("summary")
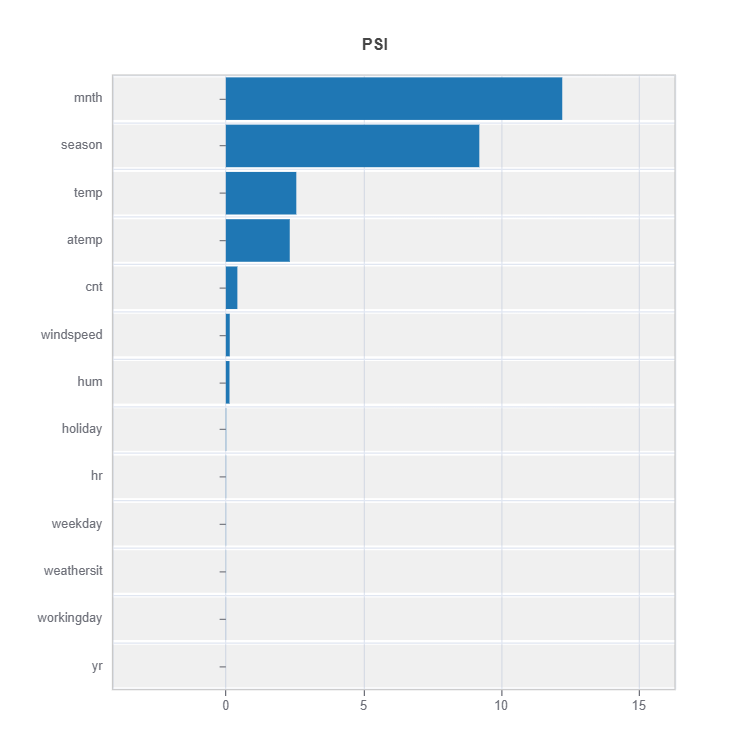
Plot the density of variables: To understand the distribution of unreliable region vs. the remaining, marginal distribution plot can be used as follows.
data_results.plot(("density", "hr"))
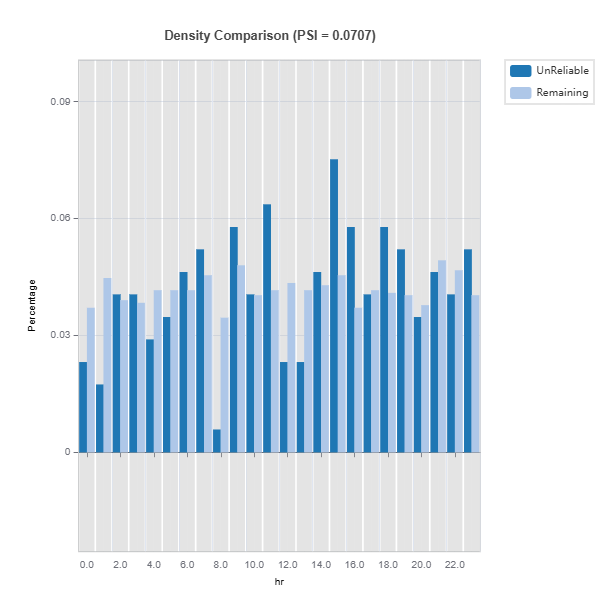
Slicing Reliability The reliability for a given feature can be analyzed through feature slicing. Below is an example for features=”hr” and metric=”width”:
results = ts.diagnose_slicing_reliability(
features="hr",
train_dataset="train",
test_dataset="test",
test_size=0.5,
metric="width",
random_state=0)
results.plot()
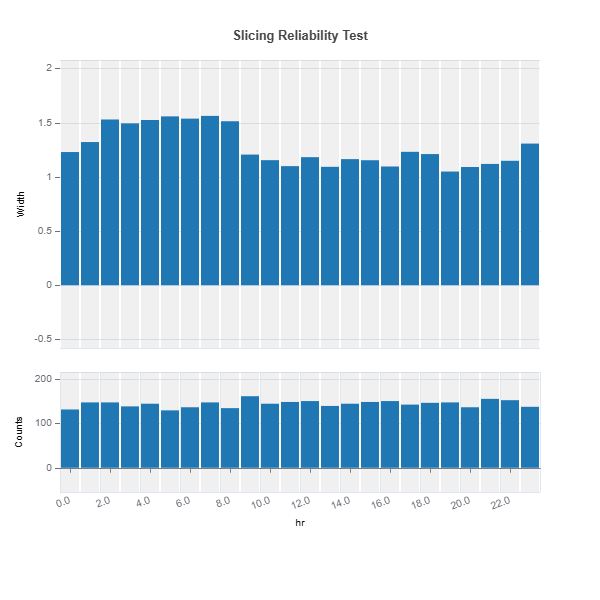
For the full list of arguments of the API see TestSuite.diagnose_slicing_reliability.
Univariate slicing for multiple features
results = ts.diagnose_slicing_reliability(
features=(("hr",), ("atemp",), ("weekday",)),
train_dataset="train",
test_dataset="test",
test_size=0.5,
metric="coverage",
random_state=0)
results.plot("hr")
results.plot("atemp")
results.plot("weekday")
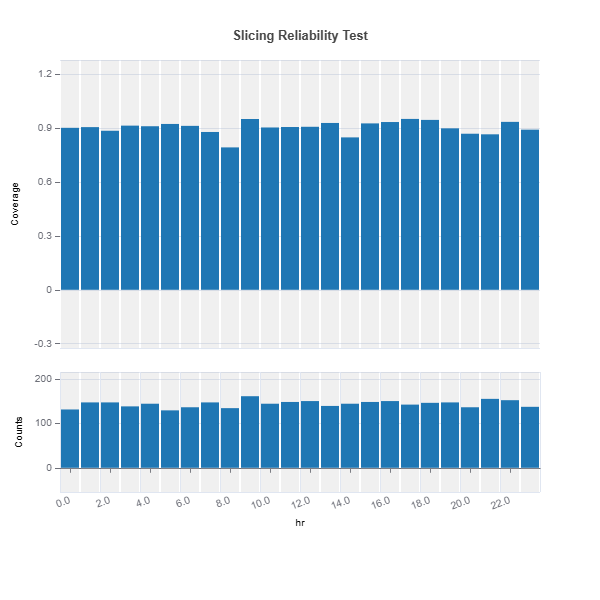
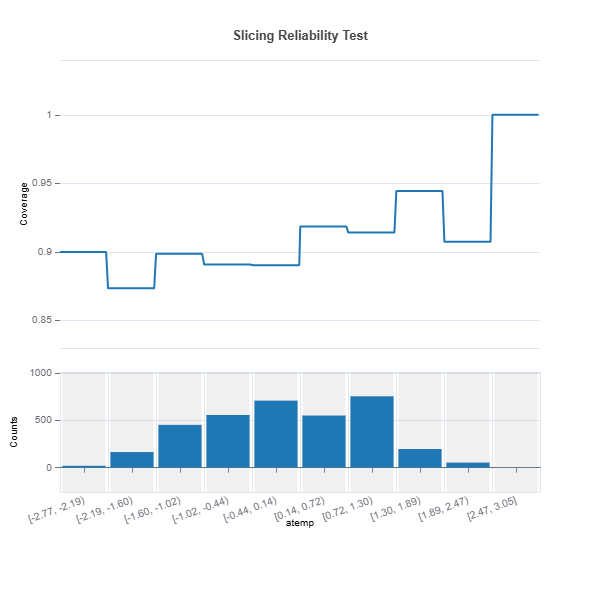
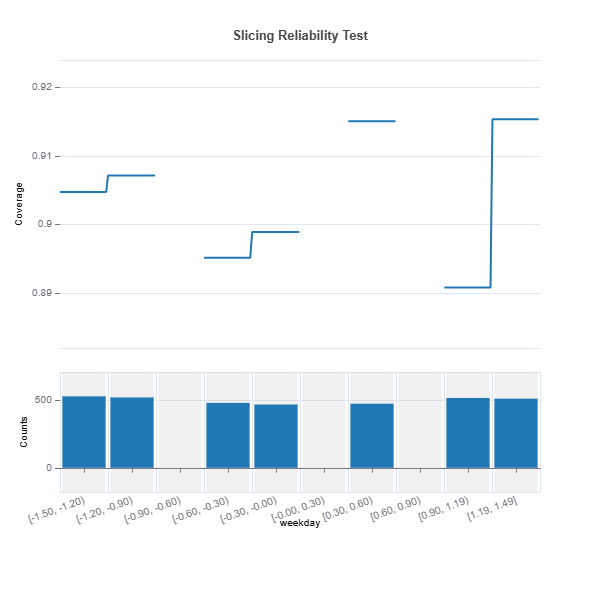
Bivariate slicing: interactions on reliability
results = ts.diagnose_slicing_reliability(
features=("hr", "atemp"),
train_dataset="train",
test_dataset="test",
test_size=0.5,
random_state=0)
results.plot()
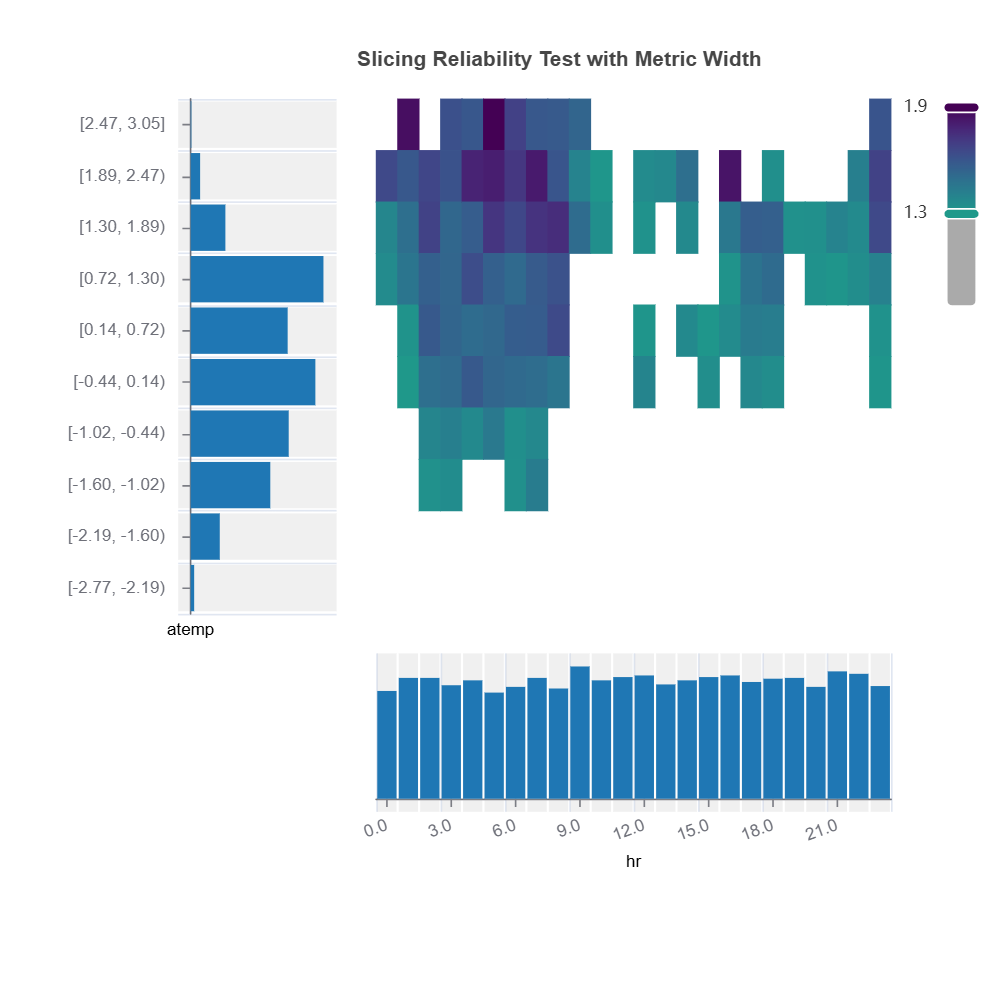
Reliability Comparison#
Reliability of several models can be compared as follows:
fsc = TestSuite(ds, models=[model_lgbm, model_xgb])
# resilreliability comparison of 2 models specified in fsc
results = fsc.compare_reliability(
train_dataset="train",
test_dataset="test",
test_size=0.5,
alpha=0.1,
max_depth=5,
random_state=0
)
results.table
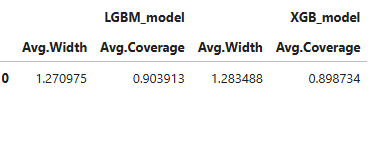
Check the API reference for detail arguments of TestSuite.compare_reliability.
The uncertainty of multiple models can be compared for each feature using variable slicing:
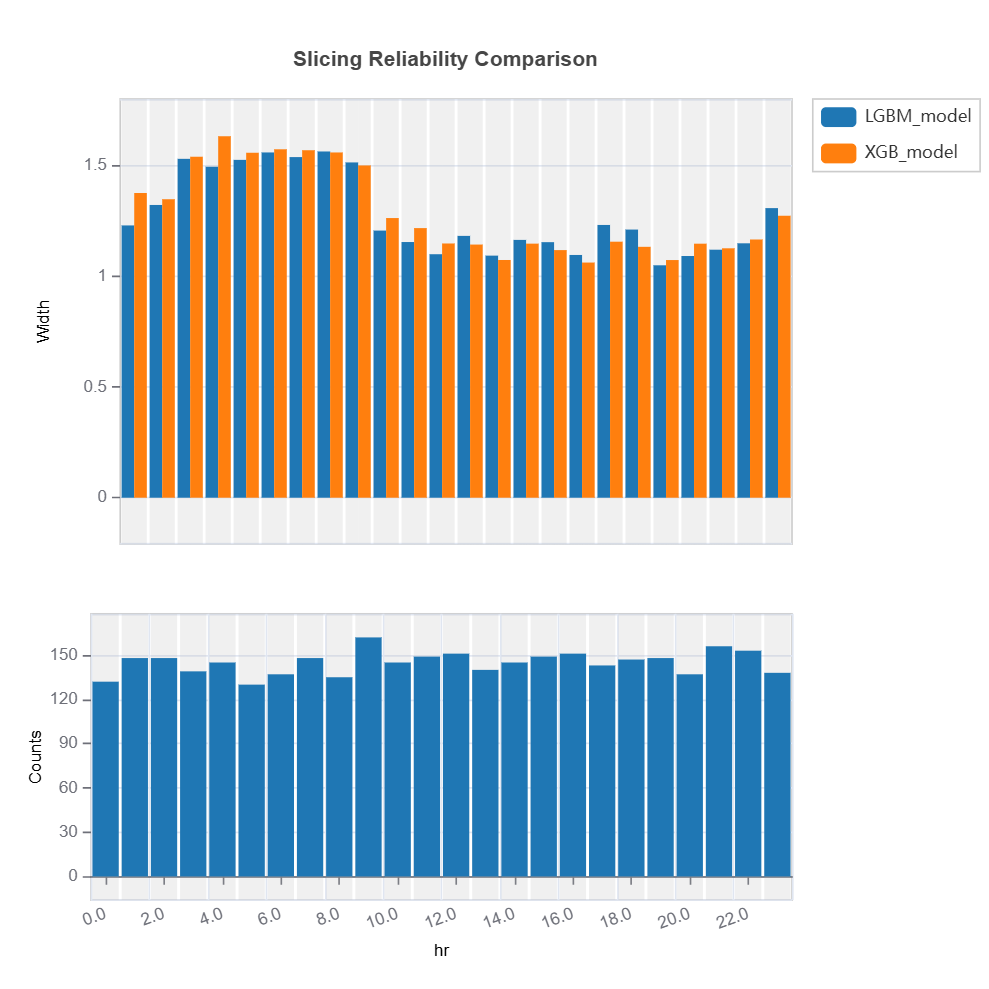
Check the API reference for detail arguments of TestSuite.compare_slicing_reliability.
Strategies for Addressing Model Weaknesses#
Data-Centric Approaches#
1. Targeted Data Augmentation:
For regions with high uncertainty or poor coverage:
Solutions:
Collect additional samples in \(R_{\text{weak}}\)
Active learning in high-uncertainty regions
2. Feature Engineering:
Based on weakness patterns:
Create interaction terms for regions with nonlinear patterns
Develop domain-specific features where coverage is poor
Transform features where uncertainty is heterogeneous
1. Local Model Enhancement:
For identified weak regions \(R_{\text{weak}}\):
Strategies:
Train specialized models for \(R_{\text{weak}}\) (segmentation)
Ensemble models with region-specific weights (Mixture of Experts)
2. Architecture Modifications:
Based on uncertainty patterns:
Add capacity in high-uncertainty regions or use alternative modeling framework
Incorporate domain knowledge via constraints
Use mixture of experts for heterogeneous regions
3. Loss Function Adjustments:
- where:
\(R(X)\) is an indicator for weak regions
\(\lambda\) balances overall performance vs local improvement
Supervised Machine Learning: Random Forest Clustering#
Prediction interval width can be used as a target clustering variable for supervised machine learning clustering using Random Forest proximity..
results = ts.diagnose_residual_cluster(
dataset="test", # dataset
response_type="pi_width", # response type
metric="MAE", # metric
n_clusters=10, # number of clusters
cluster_method="pam", # clustering method
sample_size=2000, # sample size
rf_n_estimators=100, # number of trees
rf_max_depth=5,
)
results.table #
results.plot() #
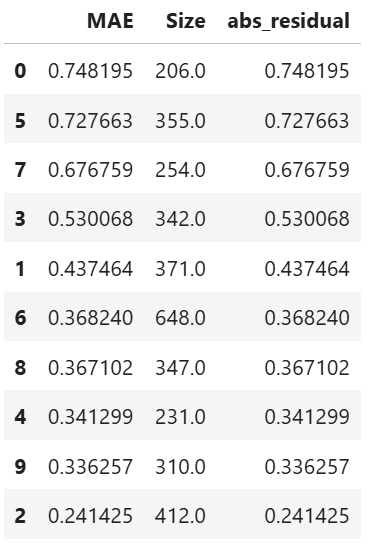
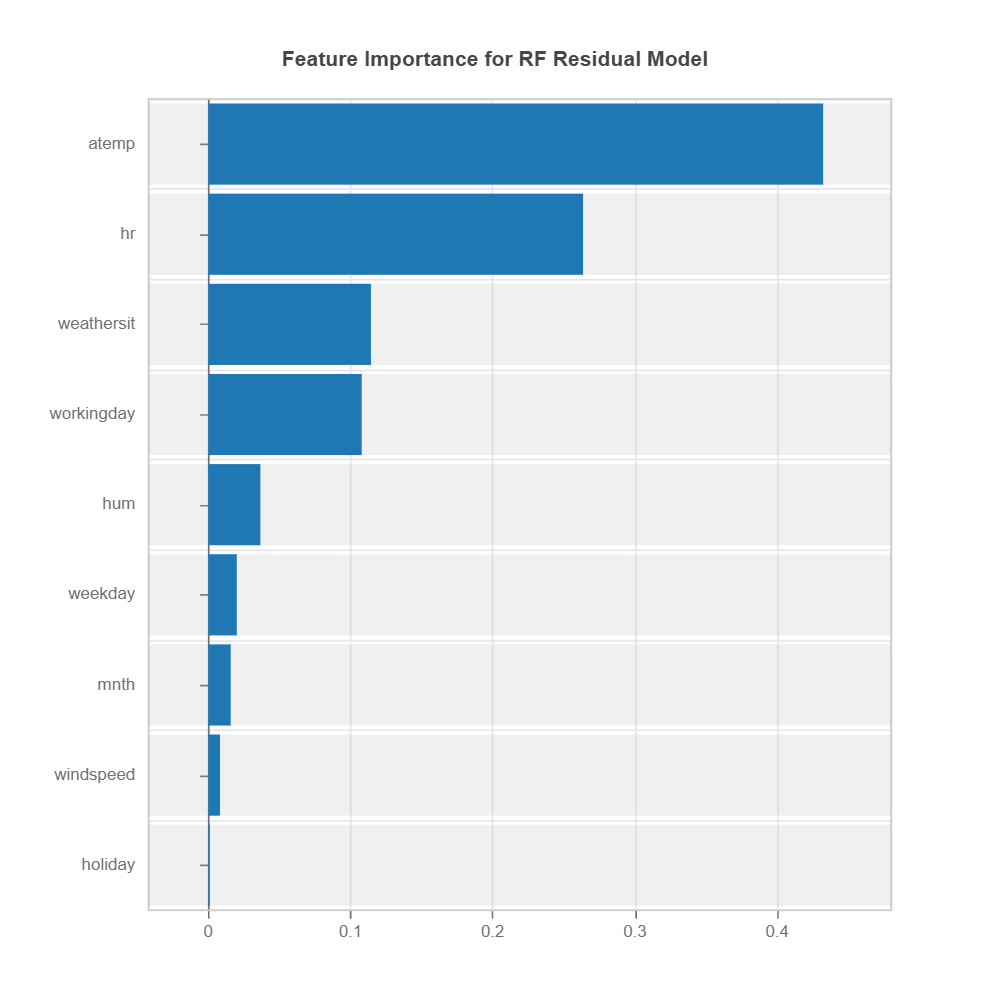
The following code shows how to identify the high-uncertainty region and interpret the results:
cluster_id = 2 # cluster id
data_results = ds.data_drift_test(
**results.value["clusters"][cluster_id]["data_info"], # use the cluster_id
distance_metric="PSI", # distance metric using PSI
psi_method="uniform", # psi method using uniform distribution
psi_bins=10 # psi bins
)
data_results.plot("summary") # plot summary of data drift test
data_results.plot(name=('density', 'atemp')) # plot density plot for feature "hr"
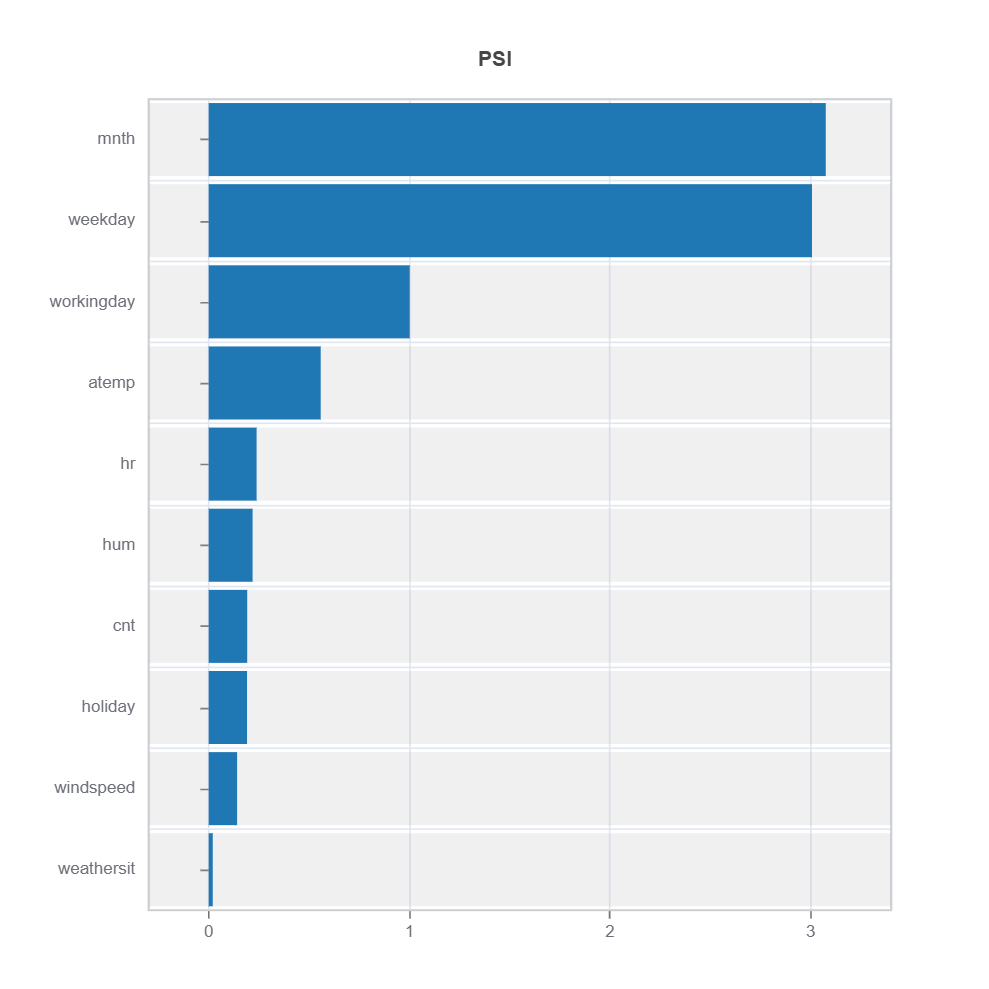
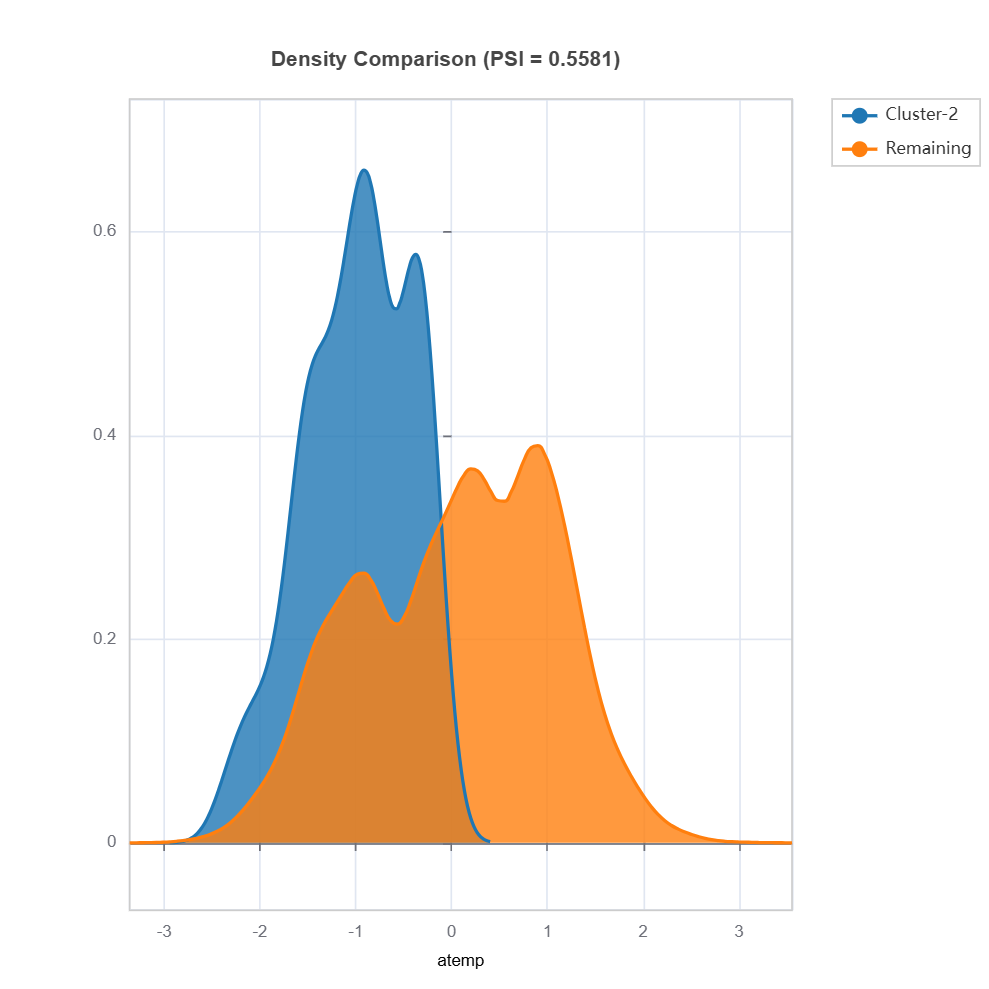