Underfitting and Overfitting#
The bias-variance tradeoff explains the relationship between a model’s ability to fit training data and its generalization to unseen data. Striking the right balance between bias and variance is critical for building robust machine learning models.
Empirical Risk and Generalization Gap#
Empirical Risk Decomposition#
The expected prediction error (empirical risk) for a model \(f̂\) at point \(x\) can be decomposed as:
- where:
\(f(x)\) is true function
\(E[f̂(x)]\) is expected prediction
\(\sigma^2\) is irreducible error
Estimation from Training and Test Errors#
Training Error:#
Underestimates true error due to fitting noise
Captures part of bias term
Does not capture variance
Test Error:#
Includes both bias and variance
Better estimate of true error
Independent of training process
Generalization Gap#
The difference between test and training error:
- This shows:
Gap directly measures overfitting
Training error underestimates true bias
Variance contributes fully to gap
Overfitting Characterization#
Based on gap magnitude:
Key insights:
Underfitting (High Bias):
Both training and testing errors are high.
The gap between them is small or negligible.
Overfitting (High Variance):
Training error is low, but testing error is significantly higher, creating a large gap.
Practical Applications#
Model Selection:
Choose models minimizing gap while maintaining acceptable training error
Use gap trends to guide complexity decisions
Training Process:
Monitor gap for early stopping
Adjust regularization based on gap
Balance model capacity against gap size
Performance Evaluation:
Compare models using both errors and gaps
Consider gap stability
Account for dataset size effects
Slicing Generalization Gap#
Slicing divides the data into subsets based on feature values, residuals, or clusters. This allows for localized analysis of model performance, helping to diagnose underfitting or overfitting in specific regions of the input space.
Define local generalization gap for region \(R\) in feature space:
where:
\(R\) is a region in feature space
\(|R_{train}|\) is number of training points in \(R\)
Weakness Detection Methods#
1. Univariate Partitioning:#
For each feature j:
where:
\(q_k\) are partitions (or quantiles) of feature \(j\)
Compute \(Gap(R_j^k)\) for each bin \(k\)
2. Multivariate Region Detection:#
Identify high-gap multivariate regions:
where:
\(q_k,q_l\) are partitions (or quantiles) of feature \(i,j\)
Compute \(Gap(R_{i,j}^{k,l})\) for each bin \(k,l\)
Identifying Problematic Regions#
Flag regions where:
where:
\(\mu_\text{gap}\) is mean gap across all regions
\(\sigma_\text{gap}\) is standard deviation of gaps
\(\beta\) is sensitivity parameter (e.g., 1.5-2)
Overfitting Slicing in MoDeVa#
Data Setup
from modeva import DataSet
## Create dataset object holder
ds = DataSet()
## Loading MoDeVa pre-loaded dataset "Bikesharing"
ds.load(name="BikeSharing")
## Preprocess the data
ds.scale_numerical(features=("cnt",), method="log1p") # Log transfomed target
ds.set_feature_type(feature="hr", feature_type="categorical") # set to categorical feature
ds.set_feature_type(feature="mnth", feature_type="categorical")
ds.scale_numerical(features=ds.feature_names_numerical, method="standardize") # standardized numerical features
ds.set_inactive_features(features=("yr", "season", "temp")) # deactivate some features
ds.preprocess()
## Split data into training and testing sets randomly
ds.set_random_split()
Model Setup
# Regression tasks using lightGBM and xgboost
from modeva.models import MoLGBMRegressor, MoXGBRegressor
# for lightGBM
model_lgbm = MoLGBMRegressor(name = "LGBM_model", max_depth=2, n_estimators=100)
# for xgboost
model_xgb = MoXGBRegressor(name = "XGB_model", max_depth=2, n_estimators=100)
Model Training
# train model with input: ds.train_x and target: ds.train_y
model_lgbm.fit(ds.train_x, ds.train_y)
model_xgb.fit(ds.train_x, ds.train_y)
Reporting and Diagnostic Setup
# Create a testsuite that bundles dataset and model
from modeva import TestSuite
ts = TestSuite(ds, model_lgbm) # store bundle of dataset and model in fs
# overfit (gap) slicing for features = season
results = ts.diagnose_slicing_overfit(
train_dataset="train",
test_dataset="test",
features="season",
method = "quantile",
metric="MAE",
threshold=0.0065)
results.table
# To visualize the results
results.plot()
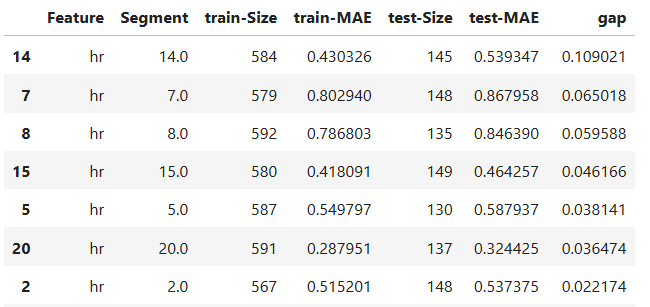
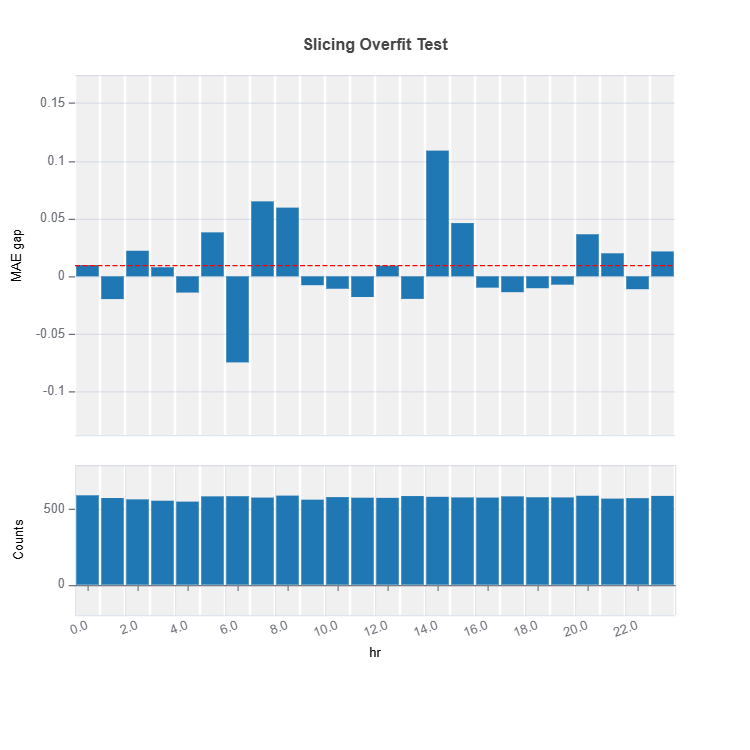
The slicing above is done using “method = quantile” binning with “threshold = 0.0065”. For the full list of arguments of the API see TestSuite.diagnose_slicing_overfit.
Retrieving samples below threshold value
from modeva.testsuite.utils.slicing_utils import get_data_info
data_info = get_data_info(res_value=results.value)["hr"]
data_info
Comparing distribution difference between below and above threshold
data_results = ds.data_drift_test(
**data_info,
distance_metric="PSI",
psi_method="uniform",
psi_bins=10)
data_results.plot("summary")
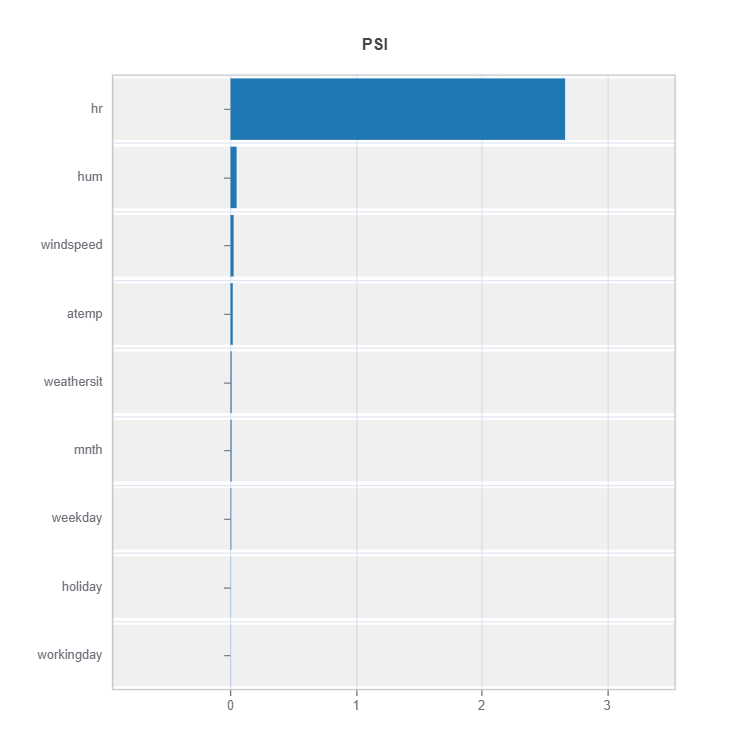
Slicing for a Set of Features with automated binning:
results = ts.diagnose_slicing_overfit(
train_dataset="train",
test_dataset="test",
features=(("hr", ), ("workingday",), ("atemp", )),
method="auto-xgb1",
metric="MAE",
threshold=0.0065)
results.table
# To visualize a single feature
results.plot(name="atemp")
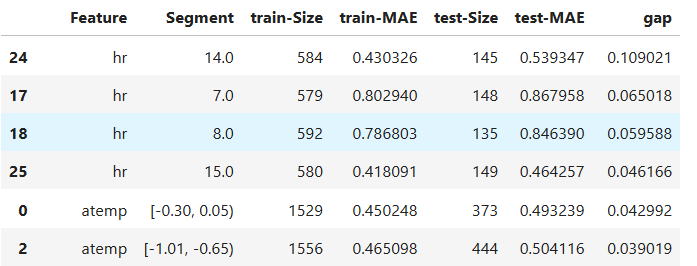
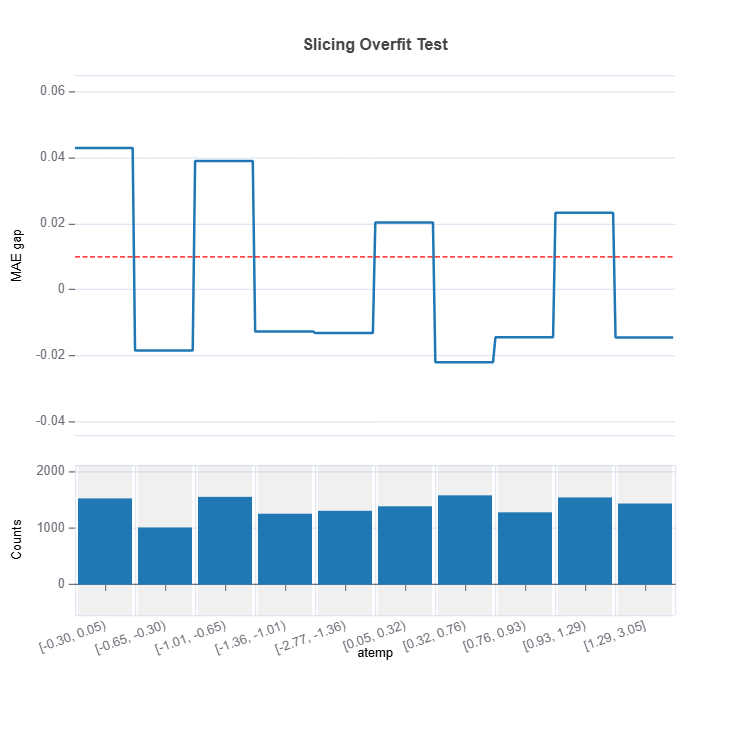
2-Feature Interaction Slicing: Two dimensional slicing
results = ts.diagnose_slicing_overfit(
train_dataset="train",
test_dataset="test",
features=("hr", "atemp"),
method="auto-xgb1",
metric="MAE",
threshold=0.0065)
results.table
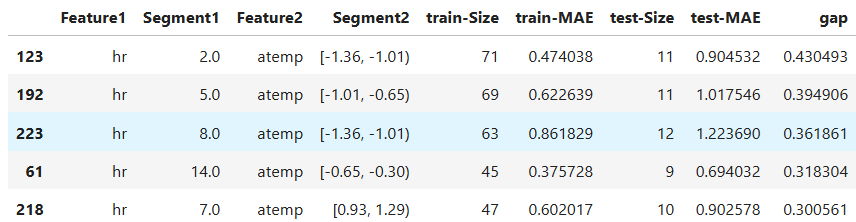
Overfit Comparison#
Slices of several models can be compared as follows:
tsc = TestSuite(ds, models=[model_lgbm, model_xgb])
results = tsc.compare_slicing_overfit(
train_dataset="train",
test_dataset="test",
features="hr",
method="quantile",
bins=10,
metric="MAE")
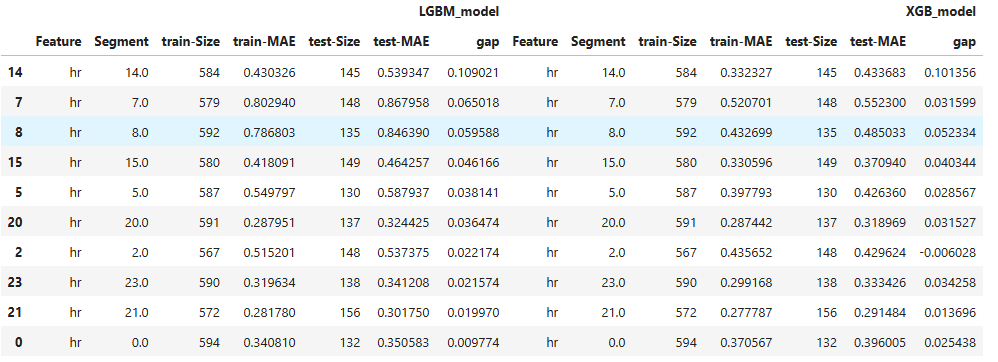
Check the API reference for detail arguments of TestSuite.compare_slicing_overfit.
Characterization of Weak Regions#
1. Data Sparsity:#
2. Complexity Measure:#
3. Uncertainty Assessment:#
Overfitting and Model Robustness#
MoDeVa provides robustness test capability. Robustness measures a model’s ability to maintain performance when subjected to input perturbations or noise. The detail description of the robustness test can be found in the robustness testing section.
The relationship between overfitting and robustness can be understood through the lens of local Lipschitz continuity:
Theoretical Framework#
1. Gradient Sensitivity:#
For an overfit model:
2. Local Curvature:#
3. Generalization Gap Connection:#
Manifestations#
Decision Boundary Complexity:
Overfit models → Complex, wiggly boundaries
More sensitive to small perturbations
Higher local Lipschitz constants
Feature Sensitivity:
Overfit models rely heavily on noise
Small changes have larger effects
Less stable predictions
Neighborhood Consistency:
Robust models → Similar predictions for similar inputs
Overfit models → More erratic local behavior
Affects adversarial robustness
Remediation Strategies for Model Weaknesses Identified by Gap Analysis#
Data-Centric Solutions#
1. Targeted Data Collection:
For regions \(R\) with large gaps:
Approaches:
Active learning in high-gap regions
Stratified sampling based on gap size
Domain expert data collection
2. Data Cleaning:
Remove noisy samples in high-gap regions
Validate labels in problematic areas
Handle outliers affecting local gaps
Feature Engineering Solutions#
1. Interaction Features:
Create new features for high-gap regions:
2. Domain-Specific Transformations:
- Examples:
Log transforms for skewed features
Binning for nonlinear relationships
Feature combinations based on domain knowledge
Apply constrainst such as monotonicity
3. Feature Selection:
Weight features by gap reduction:
Model-Centric Approaches#
1. Select alternative modeling frameworks
2. Local Model Enhancement:
3. Ensemble Strategies (Mixture of Experts):
where:
\(w_{k,i}(X)\) higher in high-gap regions
\(f_k\) specialized for different regions
Apply Mixture of Experts (MoE) model with proper regularization. The detail description of the MOE can be found in the moe section.
4. Loss Function Adjustments
1. L1/L2 Regularization:
L1 Regularization (Lasso):
- Properties:
Promotes sparsity
Helps feature selection
May be too aggressive in high-sensitivity regions
L2 Regularization (Ridge):
- Properties:
Smooths decision boundaries
More stable than L1
Might not address local sensitivity issues
2. Gap-Weighted Loss:
3. Region-Specific Penalties:
Implementation Framework#
Prioritization:
Rank regions by gap size
Assess feasibility of each solution
Consider implementation cost
Validation:
Monitor gap reduction
Check for negative side effects
Validate on holdout set
Iteration:
Start with simplest solutions
Gradually add complexity
Monitor impact on overall model
The bias-variance tradeoff highlights the balance between underfitting and overfitting. By analyzing the gap between training and testing errors and applying slicing techniques to evaluate performance across subsets, practitioners can diagnose specific issues and improve model reliability. Striking the right balance ensures optimal generalization and robust predictions for unseen data.