Mixture of Experts (MoE)#
Modern datasets—whether in finance, healthcare, or marketing—are inherently heterogeneous, comprising multiple subpopulations with distinct characteristics and drivers. Traditional “one-size-fits-all” models often fall short in such settings, leading to suboptimal predictions and reduced robustness when faced with shifts in data distribution. This motivates the need for advanced modeling approaches that achieve the following objectives:
Capturing Data Heterogeneity: By adaptively learning both local and global patterns, a model can tailor its predictions to the nuanced behaviors within different regions of the data. This adaptive learning not only improves overall predictive accuracy but also facilitates a deeper understanding of the complex interactions present in diverse datasets.
Segmented Models with Actionable Insights: Many real-world applications benefit from identifying and modeling distinct segments within the data, as each segment may be driven by unique factors. Segmenting the data enables the development of specialized models that highlight the critical drivers for each subgroup, thereby supporting targeted decision-making and interpretable results.
Enhanced Resilience Against Distribution Drift: A mixture of experts (MoE) framework naturally supports more homogeneous performance across varying data segments. By training specialized experts on segments that share similar characteristics, the model is less vulnerable to distribution drift. This segmentation ensures that even if one part of the data distribution shifts, the corresponding expert remains well-calibrated, thereby enhancing the overall model’s robustness and stability.
To address these challenges, MoDeVa provides a mixture of experts model that integrates soft clustering with expert predictors. Initially, the data is partitioned into clusters, with each sample receiving a probabilistic membership assignment across clusters. Specialized XGBoost models are then trained for each cluster using weighted samples, and their predictions are aggregated based on these membership probabilities.
This approach not only enhances predictive performance by accommodating data heterogeneity and uncovering distinct drivers but also delivers more resilient and uniformly performing models in the face of distribution drift.
The Mixture of Experts (MoE) model in MoDeVa combines multiple interpretable XGBoost models, each specializing in different regions of the feature space. By using shallow trees (depth 1 or 2) for both gating and expert models, it maintains interpretability through functional ANOVA decomposition while enabling local expertise development.
Key Benefits:
Interpretable predictions
Local specialization
Robust performance
Clear effect attribution
Model Architecture#
The model consists of three main components:
1. Gating Model:
Determines cluster memberships
Assigns sample weights
2. Expert Models:
Individual XGBoost models
Depth-restricted trees
3. Mixture Layer:
Combines expert predictions
Weights by membership probabilities
Produces final output
Mathematical Formulation#
Given a dataset \(\mathcal{D} = {(x_i, y_i)}_{i=1}^n\) where \(x_i \in \mathbb{R}^d\) represents the feature vector and \(y_i\) is the target, the model employs \(K\) experts. Each expert \(f_k(x)\) is an XGBoost model, and the overall prediction is a weighted combination of the experts’ outputs.
where each expert \(\hat{f}_k(x)\) is trained on the full dataset with samples weighted by their respective gating model \(\hat{p}_{k}(x)\).
Step-by-Step Process#
Initial Clustering: Define the number of clusters/experts (\(K\)), and compute the cluster centroids \(\{c_k\}_{k=1}^K\) using a clustering method such as k-means.
Gating Model Training: Train a gating XGBoost model (binary classification) \(\hat{p}_k(x)\) for each cluster \(k\). The gating model \(\hat{p}_{k}\) is trained on \(\{x_i, I_k(x_i)\}_{i=1}^n\), where \(I_k(x_i)\) equals to 1 when the sample belongs to the \(k\)-th cluster, otherwise 0.
Expert Training: Train an expert XGBoost model \(\hat{f}_k(x)\) for each cluster \(k\) using the full training sample. Each training sample is weighted by its corresponding membership \(\hat{p}_{k}(x)\) to focus the expert on its relevant data region.
Centroid Optimization via PSO (optional)#
Instead of using K-Means as the initial cluster, it’s also possible to treat centroids as hyperparameter, which can be directly optimized using PSO.
Particle Evaluation: For each particle (candidate centroids), retrain the gating and expert models on training data, and compute the overall validation loss.
Particle Update: Update the velocities and positions of the particles using PSO’s updating Equations.
Selection: Choose the particle with the lowest validation loss as the best candidate.
Iteration: Repeat the PSO process until the loss converges or a maximum number of iterations is reached.
MoE in MoDeVa#
Data Setup
from modeva import DataSet
## Create dataset object holder
ds = DataSet()
## Loading MoDeVa pre-loaded dataset "Bikesharing"
ds.load(name="BikeSharing")
## Preprocess the data
ds.scale_numerical(features=("cnt",), method="log1p") # Log transfomed target
ds.set_feature_type(feature="hr", feature_type="categorical") # set to categorical feature
ds.set_feature_type(feature="mnth", feature_type="categorical")
ds.scale_numerical(features=ds.feature_names_numerical, method="standardize") # standardized numerical features
ds.set_inactive_features(features=("yr", "season", "temp")) # deactivate some features
ds.preprocess()
## Split data into training and testing sets randomly
ds.set_random_split()
Model Setup
# For regression tasks
from modeva.models import MoMoERegressor
model_moe = MoMoERegressor(name="MOE_Regression", max_depth=2, n_clusters=2, n_estimators=100)
# For classification tasks
from modeva.models import MoMoEClassifier
model_moe = MoMoEClassifier(name="MOE_Classification", max_depth=2, n_clusters=2, n_estimators=100)
For the full list of hyperparameters, please see the API of MoMoERegressor and MoMoEClassifier.
Model Training
# train model with input: ds.train_x and target: ds.train_y
model_moe.fit(ds.train_x, ds.train_y)
Reporting and Diagnostics Setup
# Create a testsuite that bundles dataset and model
from modeva import TestSuite
ts = TestSuite(ds, model_moe) # store bundle of dataset and model in fs
Performance Assessment
# View model performance metrics
result = ts.diagnose_accuracy_table()
# display the output
result.table
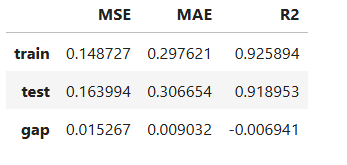
HPO on Centroids (Optional)
from modeva.models import ModelTunePSO
from modeva.models import MoMoERegressor
# define search space for centroids
n_clusters = 2
param_bounds = {'centroids': [np.repeat(ds.train_x.min(0).reshape(1, -1),
repeats=n_clusters, axis=0).ravel(),
np.repeat(ds.train_x.max(0).reshape(1, -1),
repeats=n_clusters, axis=0).ravel()]
}
# run HPO using PSO
model = MoMoERegressor(n_clusters=n_clusters, max_depth=1, n_estimators=100)
hpo = ModelTunePSO(dataset=ds, model=model)
hpo_result = hpo.run(param_bounds=param_bounds,
n_iter=10,
n_particles=5,
cv=3,
metric=("MAE", "R2")
)
# refit using best centroids and all training data
model_tuned = MoMoERegressor(**hpo_result.value["params"][0],
name="MOE-Tuned",
n_clusters=n_clusters,
max_depth=1,
n_estimators=100,
verbose=-1)
model_tuned.fit(ds.train_x, ds.train_y)
model_tuned
Interpretation: Functional ANOVA Representation#
Model interpretation is attributed to its individual expert model and functional ANOVA decomposition is similar to that of Gradient Boosted Decision Tree
Gating Decomposition#
The gating model decomposes as:
where:
\(\mu_j\) is base membership probability
\(g_{ij}(x_i)\) are main effects
\(g_{ikj}(x_i, x_k)\) are interaction effects
Expert Decomposition#
Each expert model decomposes as:
where:
\(\mu_j\) is expert’s base prediction
\(f_{ij}(x_i)\) are expert’s main effects
\(f_{ikj}(x_i, x_k)\) are expert’s interactions
Effect Attribution#
Local effects are computed for:
Gating Model:
Feature contributions to memberships (probability membership/weight)
Region assignments
Expert Models:
Feature effects on predictions
Interaction patterns
Local behavior
Global Interpretation#
The inherent interpretation of GAMI-Net includes the main effect plot, pairwise interaction plot, effect importance plot, and feature importance plot.
Feature Importance#
Feature impacts in MoE are calculated for each cluster (expert).
# Global feature importance
result = ts.interpret_fi()
# Plot the result
result.plot()
Feature important plots will be generated for each cluster (expert). The following are some examples.
For the full list of arguments of the API see TestSuite.interpret_fi.
Importance Metrics:
Based on variance of marginal effects
Normalized to sum to 1
Higher values indicate stronger influence
Accounts for feature scale differences
Effect Importance#
Impact according to functional ANOVA components (main and interaction effects) are calculated for each cluster (expert)
# Global effect importance
result = ts.interpret_ei()
# Plot the result
result.plot(n_bars = 10) # Only top 10 are displayed
For the full list of arguments of the API see TestSuite.interpret_ei.
Importance Metrics:
Based on variance of individual functional ANOVA term effects (main or interaction effect)
Higher values indicate stronger influence
Categorical Variables
One-hot encoded automatically
Can view importance per category
Interpretable through reference levels
Global Effect Plot#
Plot the main and interaction effect plot of features for each cluster (expert)
# Main effect plot of feature: "hr"
result = ts.interpret_effects(features = "hr")
# Plot the result
result.plot()
# Interaction effect plot of features: "hum" and "windspeed"
result = ts.interpret_effects(features = ("hum","windspeed"))
# Plot the result
result.plot()
To plot specific expert use:
# Main effect plot of feature: "hr"
result = ts.interpret_effects(features = "hr")
expert_name = "0" # expert name/number to plot
result.plot(expert_name)
To plot all experts to compare:
# Main effect plot of feature: "hr"
result = ts.interpret_effects(features = "hr")
expert_no = "0" # expert number to plot
result.plot(name = "all")
For the full list of arguments of the API see TestSuite.interpret_effects.
Local Interpretation#
Local interpretation is calculated for each cluster (expert).
Individual Prediction Analysis#
# Local interpretation for specific sample: sample_index = 10
result = ts.interpret_local_fi(sample_index = 10, centered = True) # local feature importance
# Plot the result
result.plot()
The local feature importances for each cluster (expert) for sample number 10.
result = ts.interpret_local_ei(sample_index = 10) # local effect importance
# Plot the result
result.plot(n_bars = 10)
For the full list of arguments of the API see TestSuite.interpret_local_fi and TestSuite.interpret_local_ei .
The weights (contribution) of experts to overall response can be checked as follows:
results = ts.interpret_local_moe_weights(sample_index = 10)
results.plot()
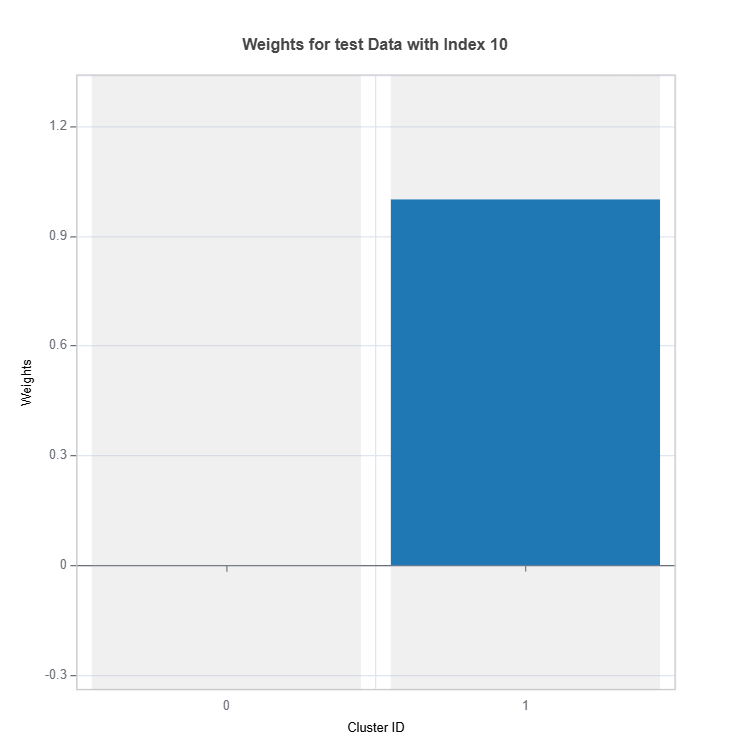
For the full list of arguments of the API see `TestSuite.interpret_local_moe_weights`_
To understand the distribution difference between a cluster (expert) to the rest, marginal distribution distance such as using PSI can be displayed as follows.
results = ts.interpret_moe_cluster_analysis()
cluster_no = 2
data_results = ds.data_drift_test(**results.value[cluster_no]["data_info"],
distance_metric="PSI",
psi_method="uniform",
psi_bins=10)
data_results.plot("summary")
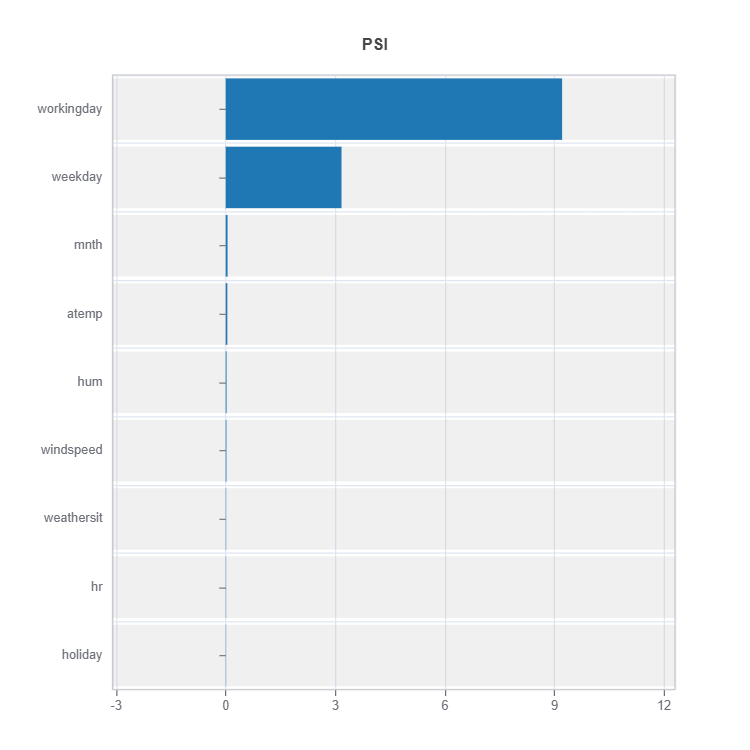
For the full list of arguments of the API see DataSet.data_drift_test.