Gradient Boosted Decision Trees#
Gradient Boosted Decision Trees (GBDT) is a powerful ensemble learning algorithm that builds a sequence of decision trees, where each subsequent tree is trained to correct the errors of its predecessors. The algorithm works by iteratively fitting trees to the negative gradient of a loss function, enabling it to handle both regression and classification tasks with remarkable accuracy. Unlike random forests which build trees independently in parallel, GBDT constructs trees sequentially, with each tree learning from previous ones.
Mathematical Formulation#
Overall Ensemble Model GBDT model can be written as sum of the initial model and the contributions of all the subsequent trees as follows:
where:
\(F_0(x)\) is the initial model (often a constant value, such as the mean in regression tasks).
\(T_m(x)\) is the decision tree (base learner) added at iteration
\(\gamma_m\) is the learning rate (or step size) controlling the contribution of each tree.
\(M\) is the total number of trees (iterations).
Pseudo-Residual Calculation At each iteration \(m\), the algorithm computes the pseudo-residuals, which are the negative gradients of the loss function \(L\) with respect to the current model’s predictions. For each sample \(x_i\) with true target \(y_i\), the pseudo-residual is given by:
where:
\(y_i\) is the true value for the \(i\) th sample.
\(F_{m-1}(x_i)\) is the prediction for the \(i\) th sample after \(m-1\) iterations.
\(r_{im}\) is the pseudo-residual for sample \(i\) at iteration \(m\)
Model Update After fitting a new decision tree \(h_m(x)\) to the pseudo-residuals, the model is updated as follows:
GBDT in MoDeVa#
MoDeVa serves as a comprehensive wrapper around the leading GBDT implementations:
XGBoost: Known for its speed and performance optimization
LightGBM: Specializes in handling large-scale datasets efficiently
CatBoost: Excels in processing categorical variables
The wrapper provides a unified interface while maintaining access to the underlying libraries’ specific capabilities and hyperparameters. This implementation choice ensures both ease of use and flexibility for advanced users.
Data Setup
from modeva import DataSet
## Create dataset object holder
ds = DataSet()
## Loading MoDeVa pre-loaded dataset "Bikesharing"
ds.load(name="BikeSharing")
## Preprocess the data
ds.scale_numerical(features=("cnt",), method="log1p") # Log transfomed target
ds.set_feature_type(feature="hr", feature_type="categorical") # set to categorical feature
ds.set_feature_type(feature="mnth", feature_type="categorical")
ds.scale_numerical(features=ds.feature_names_numerical, method="standardize") # standardized numerical features
ds.set_inactive_features(features=("yr", "season", "temp")) # deactivate some features
ds.preprocess()
## Split data into training and testing sets randomly
ds.set_random_split()
Model Setup
# For regression tasks using lightGBM, xgboost or catboost
from modeva.models import MoLGBMRegressor, MoXGBRegressor, MoCatBoostRegressor
# for lightGBM
model_gbdt = MoLGBMRegressor(name = "LGBM_model", max_depth=2, n_estimators=100)
# for xgboost
model_gbdt = MoXGBRegressor(name = "XGB_model", max_depth=2, n_estimators=100)
# for catboost
model_gbdt = MoCatBoostRegressor(name = "CBoost_model", max_depth=2, n_estimators=100)
# For classification tasks using lightGBM, xgboost or catboost
# for lightGBM
model_gbdt = MoLGBMClassifier(name = "LGBM_model", max_depth=2, n_estimators=100)
# for xgboost
model_gbdt = MoXGBClassifier(name = "XGB_model", max_depth=2, n_estimators=100)
# for catboost
model_gbdt = MoCatBoostClassifier(name = "CBoost_model", max_depth=2, n_estimators=100)
For the full list of hyperparameters, please see the API of
LightGBM regressor and classifier: MoLGBMRegressor and MoLGBMClassifier.
xgboost regressor and classifier: MoXGBRegressor and MoXGBClassifier.
CatBoost regressor and classifier: MoCatBoostRegressor and MoCatBoostClassifier.
Model Training
# train model with input: ds.train_x and target: ds.train_y
model_gbdt.fit(ds.train_x, ds.train_y)
Reporting and Diagnostics
# Create a testsuite that bundles dataset and model
from modeva import TestSuite
ts = TestSuite(dataset, model_gbdt) # store bundle of dataset and model in fs
Performance Assessment
# View model performance metrics
result = ts.diagnose_accuracy_table()
# display the output
result.table
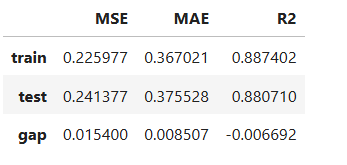
For the full list of arguments of the API see TestSuite.diagnose_accuracy_table.
Interpretability Through Functional ANOVA#
While GBDT models are typically considered black boxes, they can be made interpretable through a functional ANOVA decomposition, particularly when tree depth is constrained. The functional ANOVA framework decomposes the model into additive components [Yang2024]:
where:
\(\mu\) is the global intercept
\(f_j(x_j)\) represents main effects
\(f_{jk}(x_j, x_k)\) represents interaction effects
Special Cases with Limited Depth#
1. Depth-1 Trees (GAM Structure):
Each tree makes a single split
Results in a Generalized Additive Model (GAM)
Model captures only main effects: \(f(x) = \mu + \sum_j f_j(x_j)\)
Highly interpretable structure showing individual feature impacts
2. Depth-2 Trees (GAMI Structure):
Each tree makes up to two splits
Creates a GAM with Interactions (GAMI)
Model captures both main effects and pairwise interactions: \(f(x) = \mu + \sum_j f_j(x_j) + \sum_{j<k} f_{jk}(x_j, x_k)\)
Balances interpretability with ability to capture feature interactions
These constrained models offer several advantages:
Maintain good predictive performance
Provide clear interpretation of feature effects
Enable visualization of feature relationships
Support business decision-making with transparent logic
Functional ANOVA Decomposition Process for Tree Ensembles#
1. Aggregation Stage#
Base Representation
The tree ensemble model starts as a sum of tree structures:
\(f(x) = \sum_k \eta_k T_k(x)\)
where:
\(k\) is the number of trees
\(\eta_k\) are learning rates/weights
\(T_k\) are individual trees
Leaf Node Decomposition
Each tree is rewritten as a sum of leaf nodes:
\(f(x) = \sum_m v_m \prod_j ∈ \sum_j I(s^l_{mj} \leq x_j \lt s^u_{mj})\)
where:
\(m\) indexes leaf nodes
\(v_m\) is leaf node value \(×\) tree weight
\(S_m\) is the set of split variables in path to leaf \(m\)
\([s^l_{mj}, s^u_{mj})\) defines interval for feature \(j\)
Effect Assignment
Leaf nodes are assigned to effects based on their distinct split variables:
1. Main Effects (1 split variable):
\(f_j(x_j) = \sum_{S_m=\{j\}} v_m · I(s^l_{mj} \leq x_j \lt s^u_{mj})\)
2. Pairwise Interactions (2 split variables):
\(f_{jk}(x_j,x_k) = \sum_{S_m=\{j,k\}} v_m · I(s^l_{mj} \leq x_j \lt s^u_{mj}) · I(s^l_{mk} \leq x_k \lt s^u_{mk})\)
3. Higher-order Interactions:
Assigned based on number of distinct splits
Maximum order limited by tree depth
2. Purification Stage#
Identifiability Problem
Raw effects from aggregation may not have unique interpretation because:
Main effects can be absorbed into parent interactions
Multiple equivalent representations exist
Effects may not be mutually orthogonal
Constraint Implementation
Effects must satisfy:
\(\int f_{i_1...i_t} x_{i_1},...,x_{i_t} \, dx_k = 0, k = i_1,...,i_t\)
and
\(\int f_{i_1...i_u} (x_{i_1},...,x_{i_u}) \, \cdot \, f_{j_1...j_v} (x_{j_1},...,x_{j_v}) \, d\mathbf{x} = 0, (i_1...i_u) \ne (j_1...j_v)\)
This ensures:
All effects have zero means
Effects are mutually orthogonal
Purification Algorithm
For a pairwise interaction \(f_{jk}(x_j,x_k):\)
First Dimension:
Calculate mean along \(x_j\) dimension
Subtract mean vector from interaction matrix
Add mean to main effect \(f_k(x_k)\)
Second Dimension:
Calculate mean along \(x_k\) dimension
Subtract mean vector from interaction matrix
Add mean to main effect \(f_j(x_j)\)
Iterate until convergence:
Repeat steps 1-2 until matrix change < threshold
Results in purified interaction and updated main effects
Cascade Process
Start with highest-order interactions
Recursively process lower-order effects
Finally center main effects
Add all removed means to intercept
Global Interpretation#
The inherent interpretation of Depth-2 GBDT includes the main effect plot, pairwise interaction plot, effect importance plot, and feature importance plot.
Feature Importance
Assess overall feature impact:
# Global feature importance
result = ts.interpret_fi()
# Plot the result
result.plot()
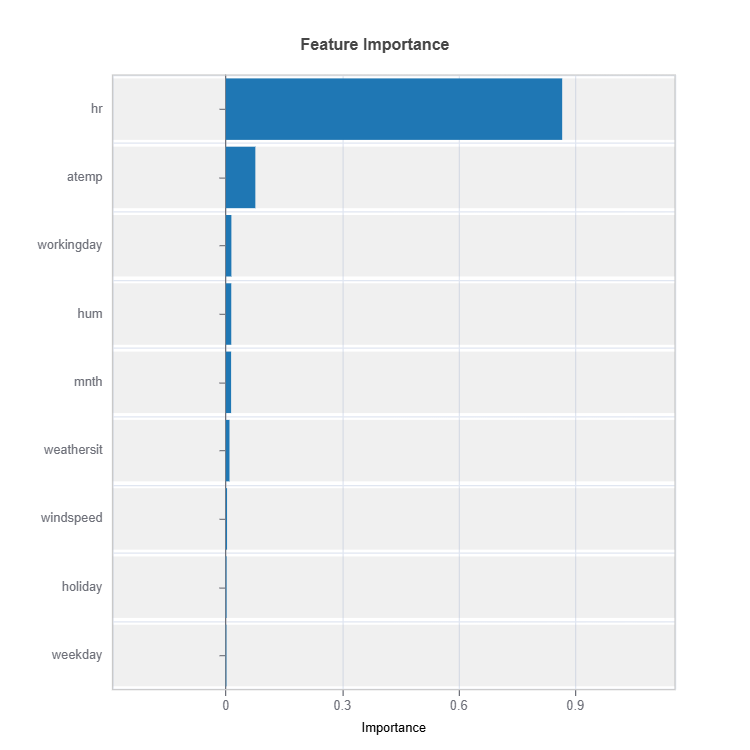
For the full list of arguments of the API see TestSuite.interpret_fi.
Importance Metrics:
Based on variance of marginal effects
Normalized to sum to 1
Higher values indicate stronger influence
Accounts for feature scale differences
Effect Importance
Assess overall impact according to functional ANOVA components: main and interaction effect
# Global effect importance
result = ts.interpret_ei()
# Plot the result
result.plot()
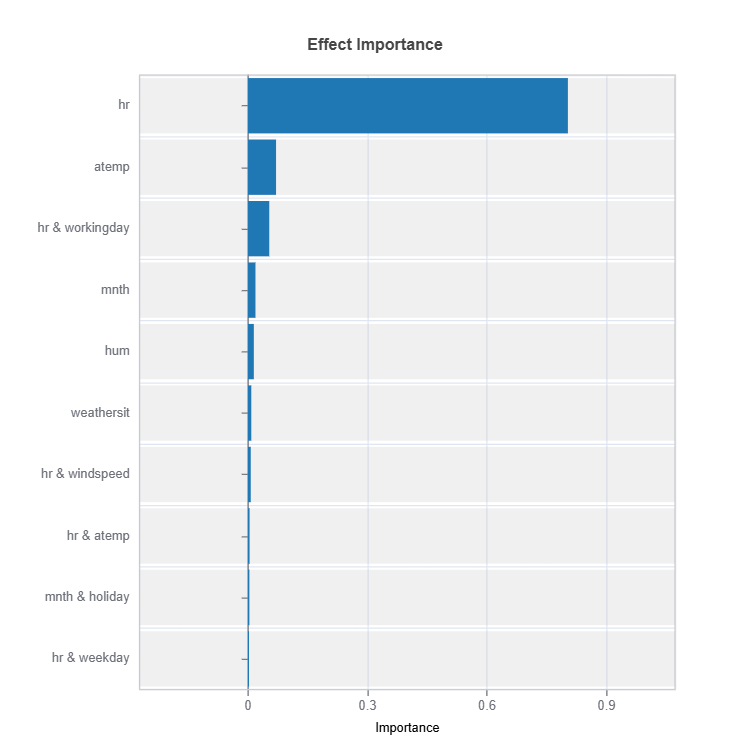
For the full list of arguments of the API see TestSuite.interpret_ei.
Importance Metrics:
Based on variance of individual functional ANOVA term effects (main or interaction effect)
Higher values indicate stronger influence
Categorical Variables
One-hot encoded automatically
Can view importance per category
Interpretable through reference levels
Global Effect Plot#
Plot the main and interaction effect plot of features
# Main effect plot of feature: "hr"
result = ts.interpret_effects(features = "hr")
# Plot the result
result.plot()
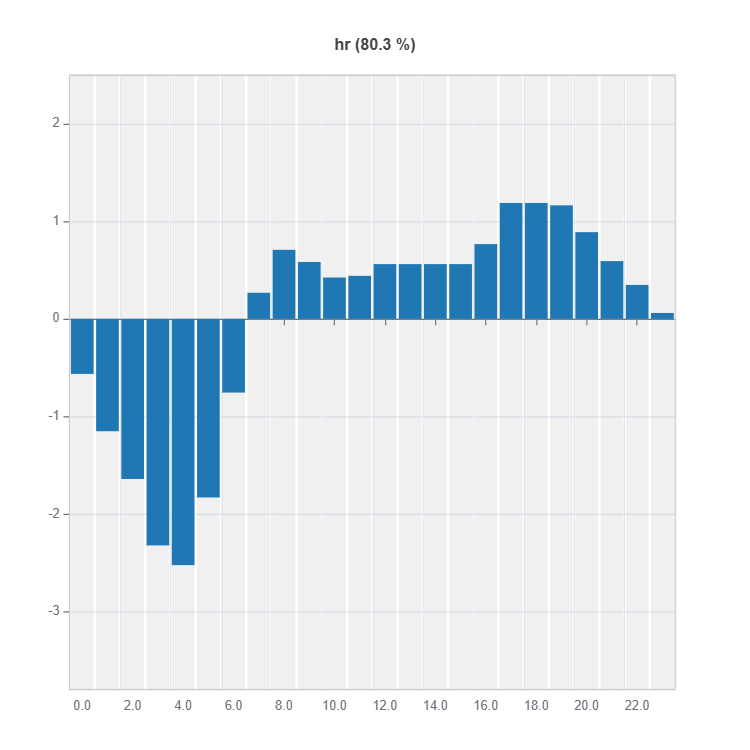
Local Interpretation#
Individual Prediction Analysis#
# Local interpretation for specific sample: sample_index = 10
result = ts.interpret_local_fi(sample_index = 10, centered = True) # local feature importance
# Plot the result
result.plot()
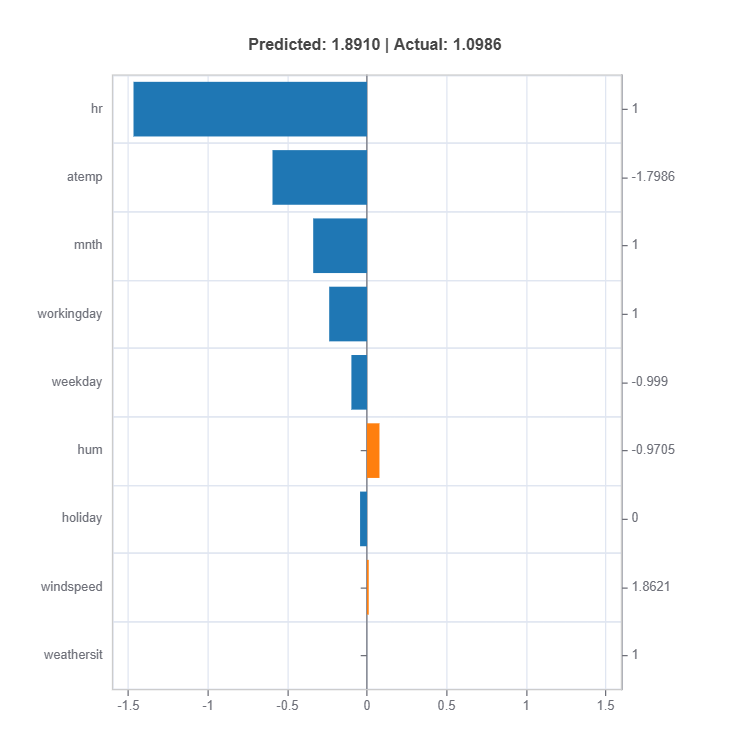
result = ts.interpret_local_ei(sample_index = 10, centered = True) # local effect importance
# Plot the result
result.plot()
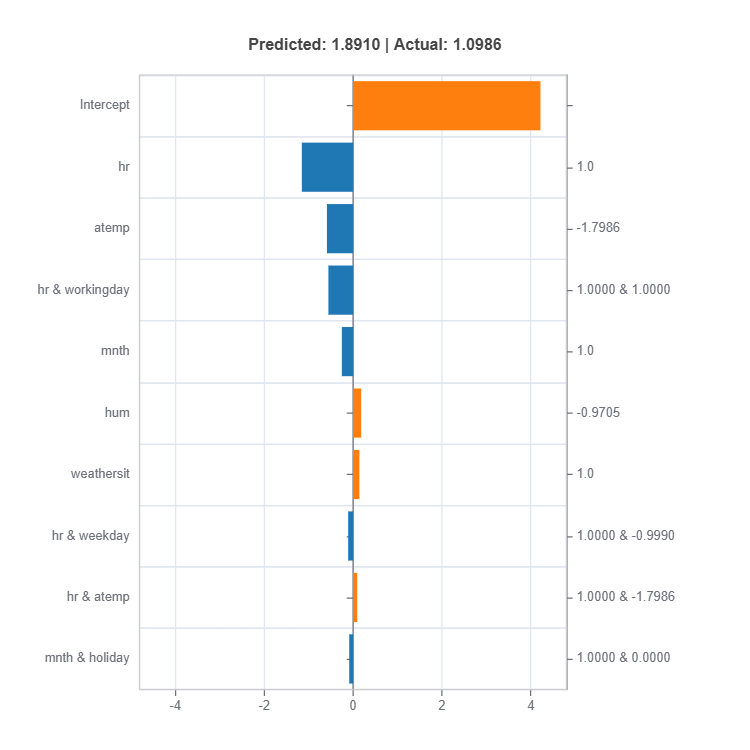
For the full list of arguments of the API see TestSuite.interpret_local_fi and TestSuite.interpret_local_ei .
Components:
Feature or Effect contributions to prediction
Feature or Effect values for the sample
Comparison to average behavior
Direction and magnitude of effects
Centering Options
Uncentered Analysis (centered=False): * Raw feature contributions * Direct interpretation * May have identifiability issues
Centered Analysis (centered=True): * Compares to population mean * More stable interpretation * Better for relative importance
Monotonicity Constraint in GBDT#
Monotonicity constraints are essential in many real-world applications where certain feature-response relationships must follow domain knowledge. For example:
Credit scoring should increase with income
Risk should decrease with credit rating
Property value should increase with square footage
xgboost regressor and classifier: MoXGBRegressor and MoXGBClassifier provide ability to control monotonicity.
Benefits#
Interpretability Enhancement#
1. Shape Functions
Smoother main effects
Reduced noise and fluctuations
Clearer global patterns
2. Local Explanations
More consistent SHAP values
Easier to explain individual predictions
Better aligned with business logic
Model Quality#
1. Robustness
Reduced overfitting
Better generalization
More reliable extrapolation
2. Performance
Often maintains or improves accuracy
More stable predictions
Better handling of sparse regions
Interaction with ANOVA Decomposition#
Main Effects#
Guarantees monotonic shape for constrained features
Preserves interpretability in functional decomposition
Simplifies effect visualization
Pairwise Interactions#
Maintains partial monotonicity
More interpretable interaction patterns
Cleaner effect separation
Empirical Results#
Monotonicity constraints often lead to minimal performance loss
Significant improvement in interpretability metrics
Better generalization in some cases
More reliable predictions in sparse data regions