Decision Tree#
A decision tree is a widely recognized machine learning algorithm that operates by recursively dividing a dataset into smaller subsets based on the most informative features. Each division is performed to optimize predictive accuracy for the target response variable. This hierarchical splitting creates a tree structure that is easy to interpret, making decision trees a popular choice for both regression and classification tasks.
Implementation Details
MoDeVa’s Tree implementation serves as a wrapper around scikit-learn’s decision tree models, providing:
Consistent API across all MoDeVa modeling frameworks
Direct access to scikit-learn’s robust implementations
Maintained compatibility with scikit-learn parameters
The underlying scikit-learn models include:
sklearn.tree.DecisionTreeRegressor
- For regression taskssklearn.tree.DecisionTreeClassifier
- For classification tasks
All arguments available in scikit-learn’s API are preserved and can be passed directly through MoDeVa’s interface. This ensures users familiar with scikit-learn can leverage their existing knowledge while benefiting from MoDeVa’s enhanced interpretation capabilities.
Decision Tree in MoDeVa#
Data Setup
from modeva import DataSet
# Create dataset object holder
ds = DataSet()
# Loading MoDeVa pre-loaded dataset "Bikesharing"
ds.load(name="BikeSharing") # Changed dataset name
# Split data into training and testing sets randomly
ds.set_random_split()
ds.set_target("cnt") # set the target variable
ds.scale_numerical(features=("cnt",), method="log1p") # scale the target variable
ds.preprocess() # preprocess the data
Model Setup
# For regression tasks
from modeva.models import MoDecisionTreeRegressor
model_dtree = MoDecisionTreeRegressor(name="Tree", max_depth=5)
# For classification tasks
from modeva.models import MoDecisionTreeClassifier
model_dtree = MoDecisionTreeClassifier(name = "Tree", max_depth=5)
For the full list of hyperparameters, please see the API of MoDecisionTreeRegressor and MoDecisionTreeClassifier.
Model Training
# train model with input: ds.train_x and target: ds.train_y
model_dtree.fit(ds.train_x, ds.train_y)
Reporting and Diagnostics
# Create a testsuite that bundles dataset and model
from modeva import TestSuite
ts = TestSuite(ds, model_dtree) # store bundle of dataset and model in ts
Performance Assessment
# View model performance metrics
result = ts.diagnose_accuracy_table()
# display the output
result.table
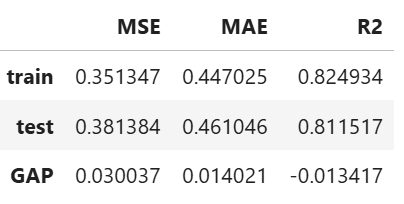
Global Interpretation#
# Global interpretation
results = ts.interpret_global_tree()
results.plot()
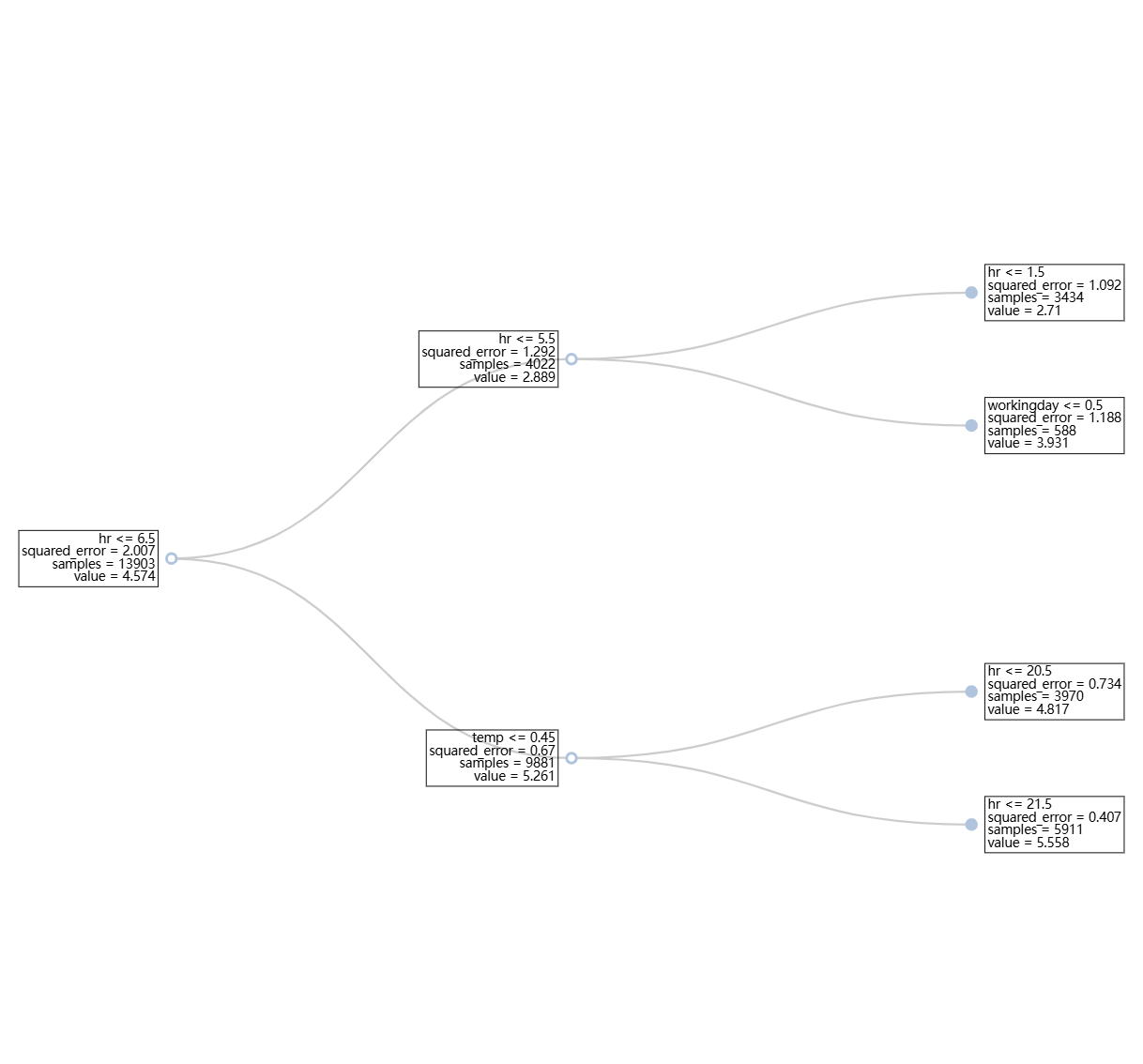
For the full list of arguments of the API see TestSuite.interpret_global_tree.
This generates a visualization of the complete decision tree model structure showing all nodes and splits in the decision tree model, providing a global view. Each box in the diagram represents a node in the decision tree. It includes information such as the node ID, the splitting rule used to split the dataset, the criterion value, the sample size, and the average value of the response.
Local Interpretation#
# # Local interpretation for specific sample: sample_index = 0
results = ts.interpret_local_tree(sample_index=0)
results.plot()
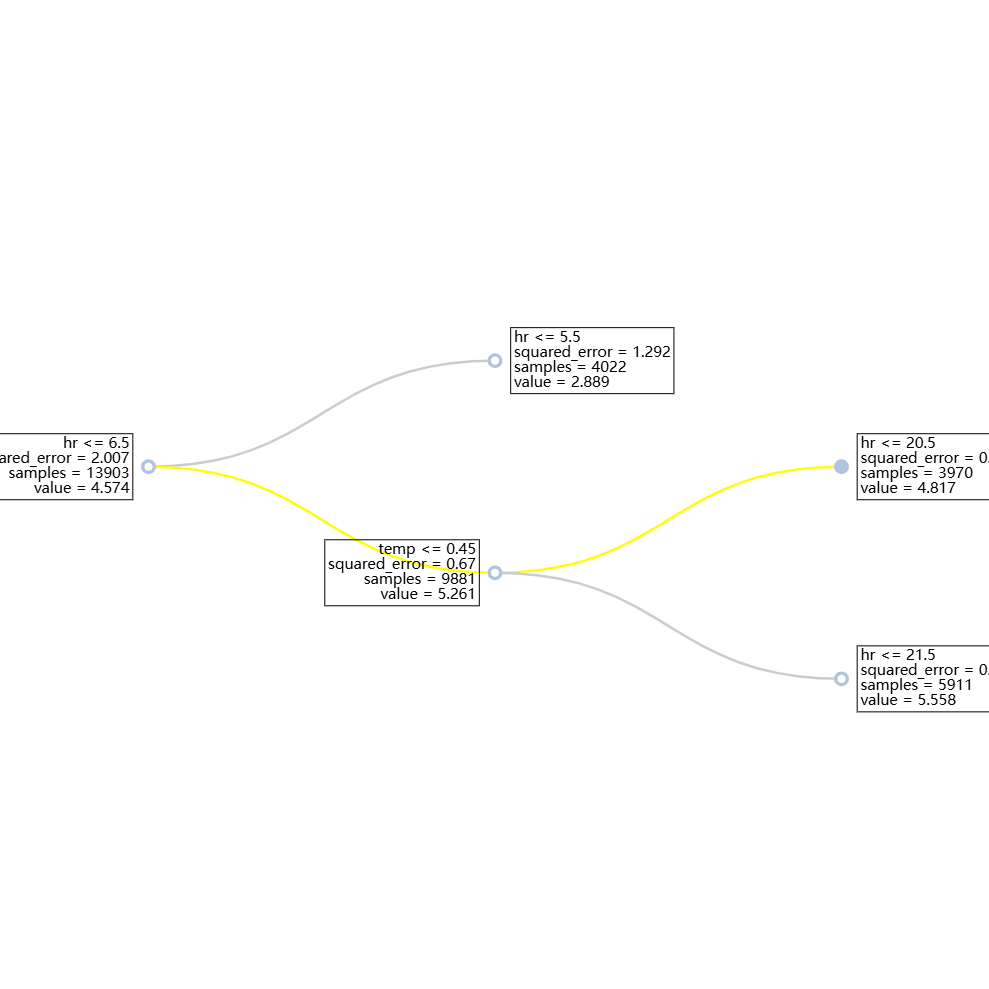
For the full list of arguments of the API see TestSuite.interpret_local_tree.
This generates a visualization of the decision path for a specific sample through the decision tree by highlighting the specific nodes and path traversed when classifying/predicting a single sample.